Driving the biological transformation with automation
Thursday, August 29th, 2024
Economists have been predicting the end of linear growth for some time. Further development towards a circular economy is the next big goal, as it offers new growth potential. And nature is an excellent role model in this regard because it knows no waste. For Festo, integrating biology as a basis for ongoing development in automation is particularly promising. It can be used to transform a biological cell into the smallest factory of the future.
To do this, we need to understand the biological processes and create optimal conditions to grow or manipulate different organisms. By using AI and quantum sensors to analyze the state of growth, we can then control and optimally monitor various parameters.
The aim of our work in this area is to create solutions that are real alternatives to today’s established chemical processes, which often require a lot of heat and pressure and are mostly based on fossil raw materials.
Using the example of photobioreactors for the production of algae and the conversion of hydrogen into alternative energy sources, Festo shows how automation can contribute to a significant increase in efficiency in the future and thus to sustainable business.
After studying mechanical engineering at the University of Aachen, Dr. Kriwet spent several years as research associate at The Institute for Machine Tools and Production Technology of the TU Berlin, followed by the Institute for Management and Technology IMT in Berlin. In 1995 Dr. Kriwet joined Festo and contributed significantly to the development of the new founded Cybernetic division. His mission here was the transformation of the component business of Festo to a solution business, creating customer advantages through ease of integration. In 2009 Dr. Kriwet was appointed to the Management Board of the Festo AG for Region and Sales Europe, followed by the responsibility for Global Sales in 2013. Since September 2022 Dr. Kriwet has been the Chief Technology Officer of Festo SE & Co. KG and is responsible for Global Research and Development in this area. He is driving the integration of electronic controls, sensors, communication, and Software with traditional mechanical motion components, enhancing customer value through data by reducing energy consumption, prolonging lifetime, and increasing OEE.
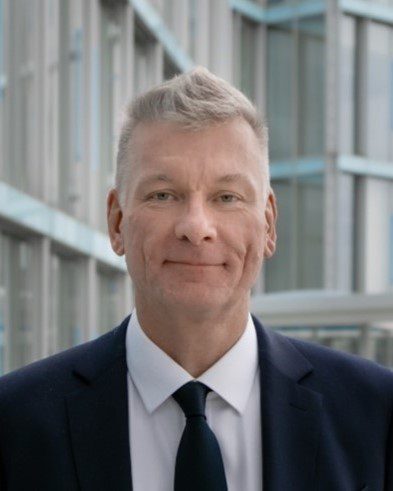
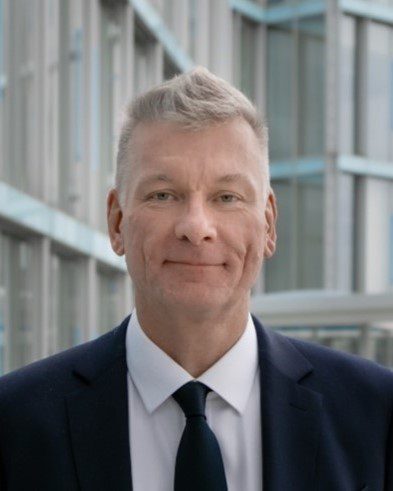
Ansgar Kriwet
Member of the Management Board Research and Development
Festo AG & Co. KG, Germany.
Event-based reinforcement learning for cyber-physical energy systems – smart buildings, smart grid, and smart cities
Friday, August 30th, 2024
Cyber physical energy system (CPES) is where information and energy merges together to improve the overall system performance including economic, comfort, and safety aspects. Artificial intelligence which are enabled by internet of things (IoT), big data, and cloud computing, has a big role in the optimization of CPES. In this talk, we focus on event-based reinforcement learning (eRL) which makes decisions according to events instead of states. This method provides a scalable solution for large-scale multi-stage decision making problem in which an accurate model may not be available. The performance of this method will be demonstrated by examples in smart buildings, smart micro-grid of buildings, and smart cities, and in particular on the problem of stochastic matching between the renewable power generation and the uncertain charging demand from the plug-in electric vehicles (PHEVs) in a city. We will also discuss extensions of this method to distributed optimization. We hope this work sheds light to the optimization of CPES.
Qing-Shan Jia received the B.S. degree in automation in July 2002 and the Ph.D. degree in control science and engineering in July 2006, both from Tsinghua University, Beijing, China. He is a Full Professor in the Center for Intelligent and Networked Systems (CFINS), Department of Automation, Tsinghua University, where he currently chairs the institute of systems engineering. He was the vice dean of Tsinghua Global Innovation eXchange (GIX) institute from 2016 to 2019 and the vice chair of Department of Automation at Tsinghua University from 2015 to 2018. He was a postdoc at Harvard University in 2006, a visiting scholar at the Hong Kong University of Science and Technology in 2010, and at Laboratory for Information and Decision Systems, Massachusetts Institute of Technology in 2013. His research interest is to develop an integrated data-driven, statistical, and computational approach to find designs and decision-making policies which have simple structures and guaranteed good performance. His work relies on strong collaborations with experts in manufacturing systems, energy systems, autonomous systems, and smart cities. He is currently the executive editor-in-chief of Results in Control and Optimization, and an associate editor (AE) of Science China Information Sciences. He was an AE of IEEE Transactions on Automatic Control (2015-2021), IEEE Control Systems Letters (2019-2021), IEEE Transactions on Automation Science and Engineering (2012-2017), and Discrete Event Dynamic Systems – Theory and Applications (2012-2016). He served the Discrete Event Systems Technical Committee chair in IEEE Control Systems Society (2012-2015), the co-chair for Smart Buildings Technical Committee in IEEE Robotics and Automation Society (2012-2021), the Control for Smart Cities Technical Committee chair in International Federation of Automatic Control (2017-2023), and the Beijing Chapter Chair of IEEE Control Systems Society (2012-2023). He is a founding chair of the Technical Committee on Machine Learning for Automation in IEEE Robotics and Automation Society (since 2023). He has been a member of the Chinese Automation Association Technical Committee on Control Theory (since 2018) and the Chinese Automation Association Technical Committee on Information Security of Industrial Systems (since 2016).
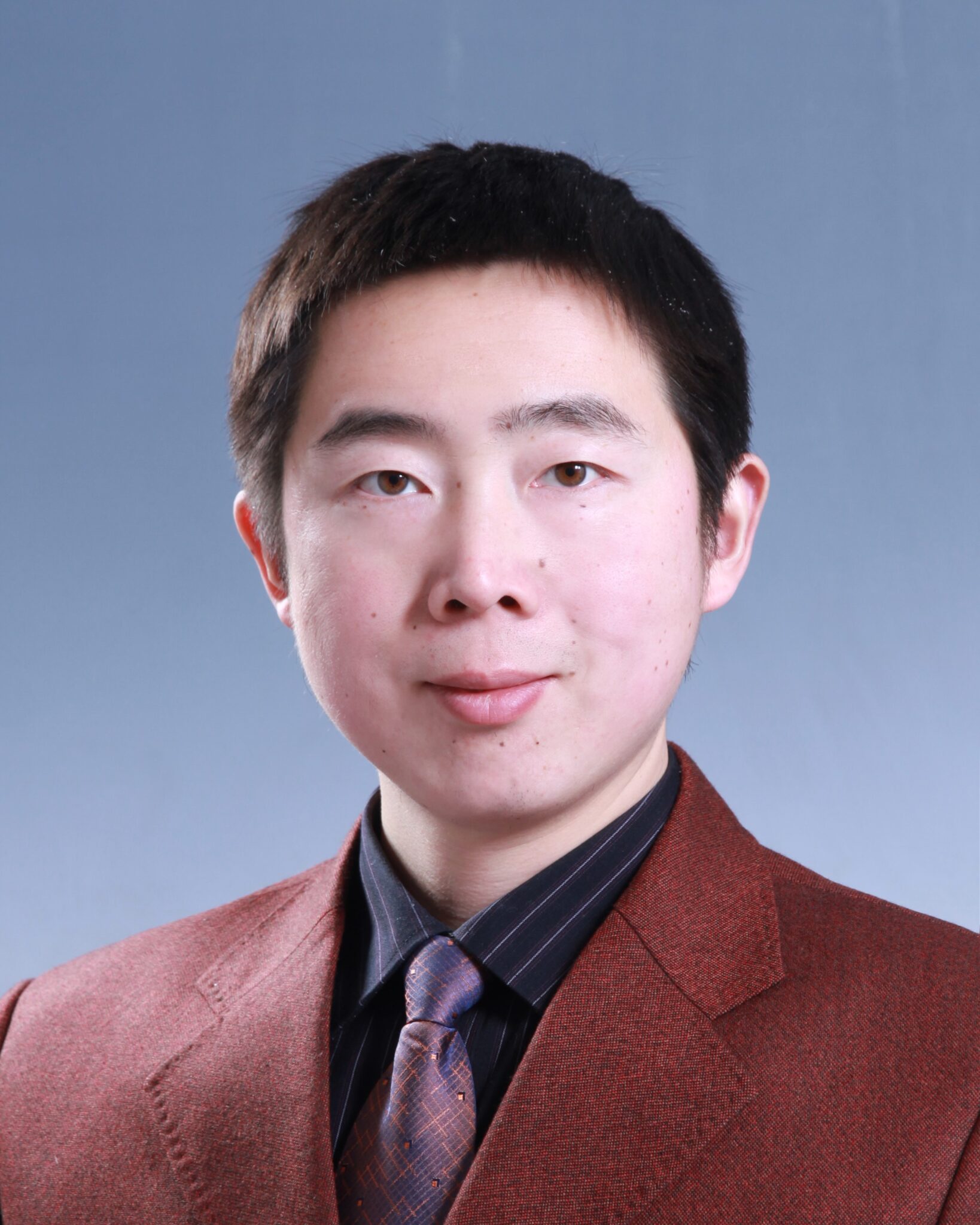
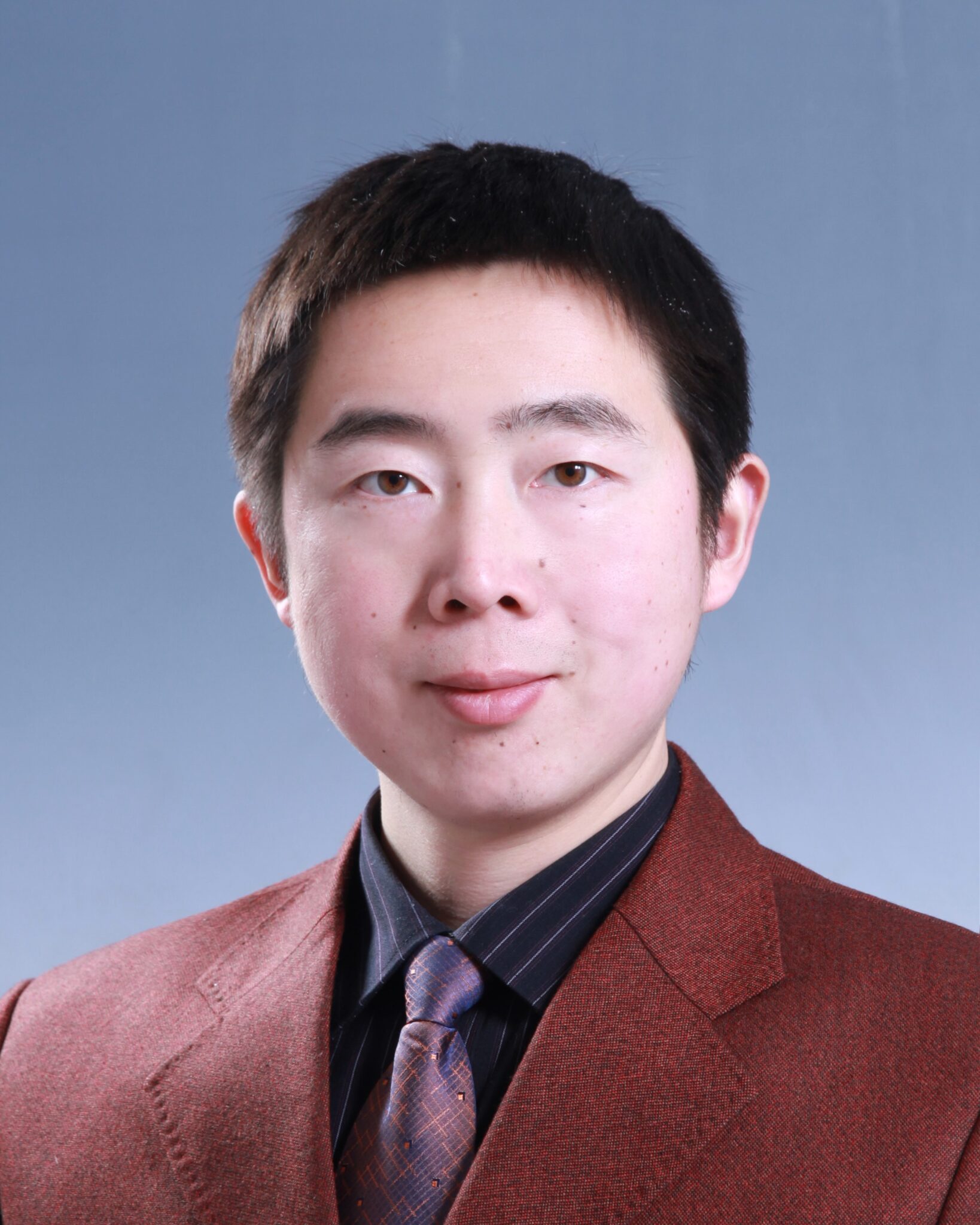
Qing-Shan Jia
Professor, Center for Intelligent and Networked Systems, Tsinghua University, China.
Machine learning methods for real-time robot control with theoretical guarantees
Saturday, August 31st , 2024
Deployment of robots in human-inhabited environments requires allowing robots to react rapidly, robustly and safely to changes in the environment. Recent advances in machine learning to analyze and model a variety of data offer powerful solutions for real-time control. For these techniques to be efficiently deployed and endorsed, they must be accompanied with explicit guarantees on the learned model.
This talk will give an overview of a variety of methods to endow robots with the necessary reactivity to adapt their path at time-critical situations. The learned control laws are accompanied by theoretical guarantees for stability and boundedness. Paucity of data is a reality in many robotics tasks. I will present methods by which robots can learn control laws from only a handful of examples, while generalizing to the entire state space. I will present a variety of applications, from dynamic manipulation in interaction with humans to reactive navigation in crowded pedestrian environments.
Aude Billard is full professor, head of the LASA laboratory and the Associate Dean for Education in School at the School of Engineering at the Swiss Institute of Technology Lausanne (EPFL). Prof Billard currently serves as the President of the IEEE Robotics and Automation Society, director of the ELLIS Robot Learning Program and co-director of the Robot Learning Foundation, a non-profit corporation that serves as the governing body behind the Conference on Robot Learning (CoRL), and leads the Innovation Booster Robotics, a program funding technology transfer in robotics and powered by the Swiss Innovation Agency, Innosuisse.
Prof Billard holds a BSc and MSc in Physics from EPFL and a PhD in Artificial Intelligence from the University of Edinburgh. Prof Billard is an IEEE Fellow and the recipient of numerous recognitions, among which the Intel Corporation Teaching award, the Swiss National Science Foundation career award, the Outstanding Young Person in Science and Innovation from the Swiss Chamber of Commerce, the IEEE RAS Distinguished Award, and the IEEE-RAS Best Reviewer Award. Dr. Billard was a plenary speaker at major robotics, AI and Control conferences (ICRA, AAAI, CoRL, HRI, CASE, ICDL, ECML, L4DC, IFAC Symposium, ROMAN, Humanoids and many others) and acted on various positions on the organization committee of numerous International Conferences in Robotics. Her research spans the fields of machine learning and robotics with a particular emphasis on fast and reactive control and on safe human-robot interaction. This research received numerous best conference paper awards, as well as the prestigious King-Sun Fu Memorial Award for the best IEEE Transaction in Robotics paper, and is regularly featured in premier venues (BBC, IEEE Spectrum, Wired).
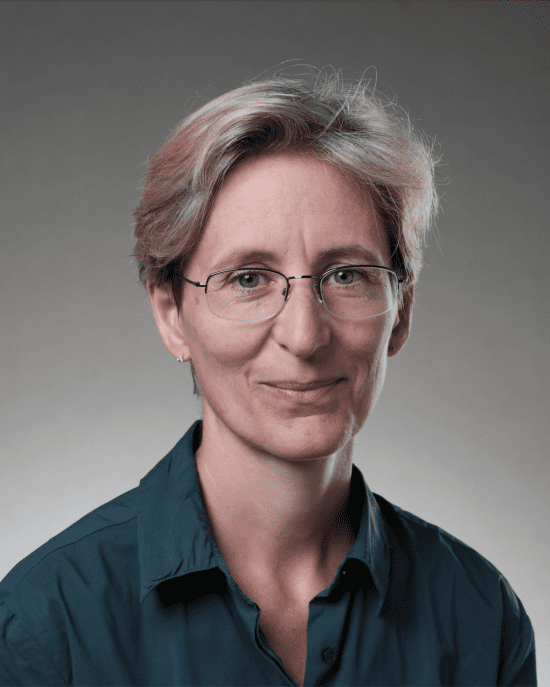
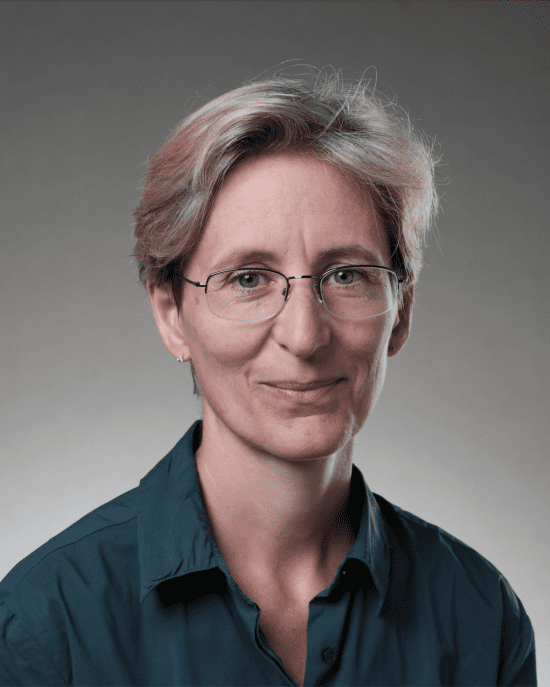
Aude Billard
Full professor and head of the LASA laboratory at the School of Engineering at the Swiss Institute of Technology Lausanne (EPFL), Switzerland.
Measuring and enhancing network resilience: metrics, learning, and defense strategies
Sunday, September 1st , 2024
Resilience, understood as the ability of a network to carry out ist goals under adversarial attacks and unexpected failures, is critical for autonomy. Despite important advances in the design of distributed coordination and decision-making algorithms, multi-agent networks have proven fragile to targeted attacks. Novel theories and tools are therefore needed to guarantee resiliency of these systems, being the development of notions and techniques that characterize network critical resilience. However, obtaining such characterizations is difficult as resilience and performance are a complex function of the network’s and adversary’s capabilities, knowledge, resources, and the network interconnection structure. At the same time, we also need novel design methodologies that can protect multi-agent networks and adaptively manage their interconnection over time to achieve performance guarantees. In this talk, we present our recent progress in these directions including algorithmic methods for metric computation, data-driven algorithms for multi-agent learning, and defense strategies.
Sonia Martínez is a Full Professor at the Department of Mechanical and Aerospace Engineering at the University of California, San Diego and a Jacobs Faculty Scholar. Prof. Martínez received her B.S. degree from the Universidad de Zaragoza, Spain in 1997, and her Ph.D. degree in Engineering Mathematics from the Universidad Carlos III de Madrid, Spain, in May 2002. Following a year as a Visiting Assistant Professor of Applied Mathematics at the Technical University of Catalonia, Spain, she obtained a Postdoctoral Fulbright Fellowship and held appointments at the Coordinated Science Laboratory of the University of Illinois, Urbana-Champaign during 2004, and at the Center for Control, Dynamical systems and Computation (CCDC) of the University of California, Santa Barbara during 2005. From January 2006 to June 2010, she was an Assistant Professor with the department of Mechanical and Aerospace Engineering at the University of California, San Diego. From July 2010 to June 2014, she was an Associate Professor with the department of Mechanical and Aerospace Engineering at the University of California, San Diego. Dr Martínez’ research interests include networked control systems, multi-agent systems, and nonlinear control theory with applications to robotics, cyber-physical systems, and natural/social networks. In particular, she has focused on the modeling and control of robotic sensor networks, the development of distributed coordination algorithms for groups of autonomous vehicles, and the geometric control of mechanical systems. For her work on the control of underactuated mechanical systems she received the Best Student Paper award at the 2002 IEEE Conference on Decision and Control. She was the recipient of a NSF CAREER Award in 2007. For the co-authored papers “Motion coordination with Distributed Information,” and “Tutorial on dynamic average consensus: The problem, its applications, and the algorithms”, she received respectively the 2008 and 2021 Control Systems Magazine Outstanding Paper Award. She was named IEEE Fellow, class of 2018 for her work on Geometric Mechanics and Multi-Agent systems. She is a Senior Editor of Automatica and the Editor in Chief of the CSS publication IEEE Open Journal of Control Systems (IEEE OJCS).
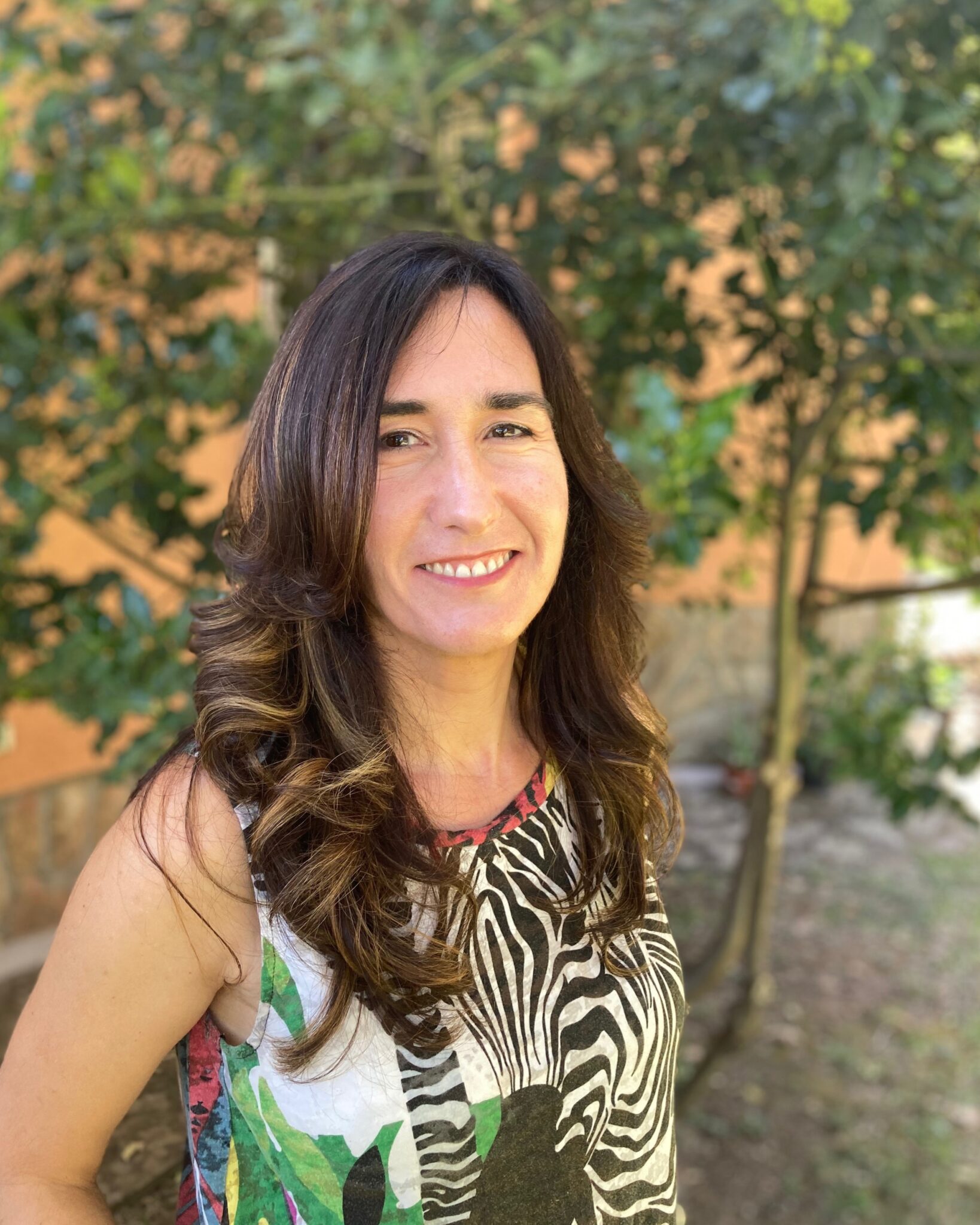
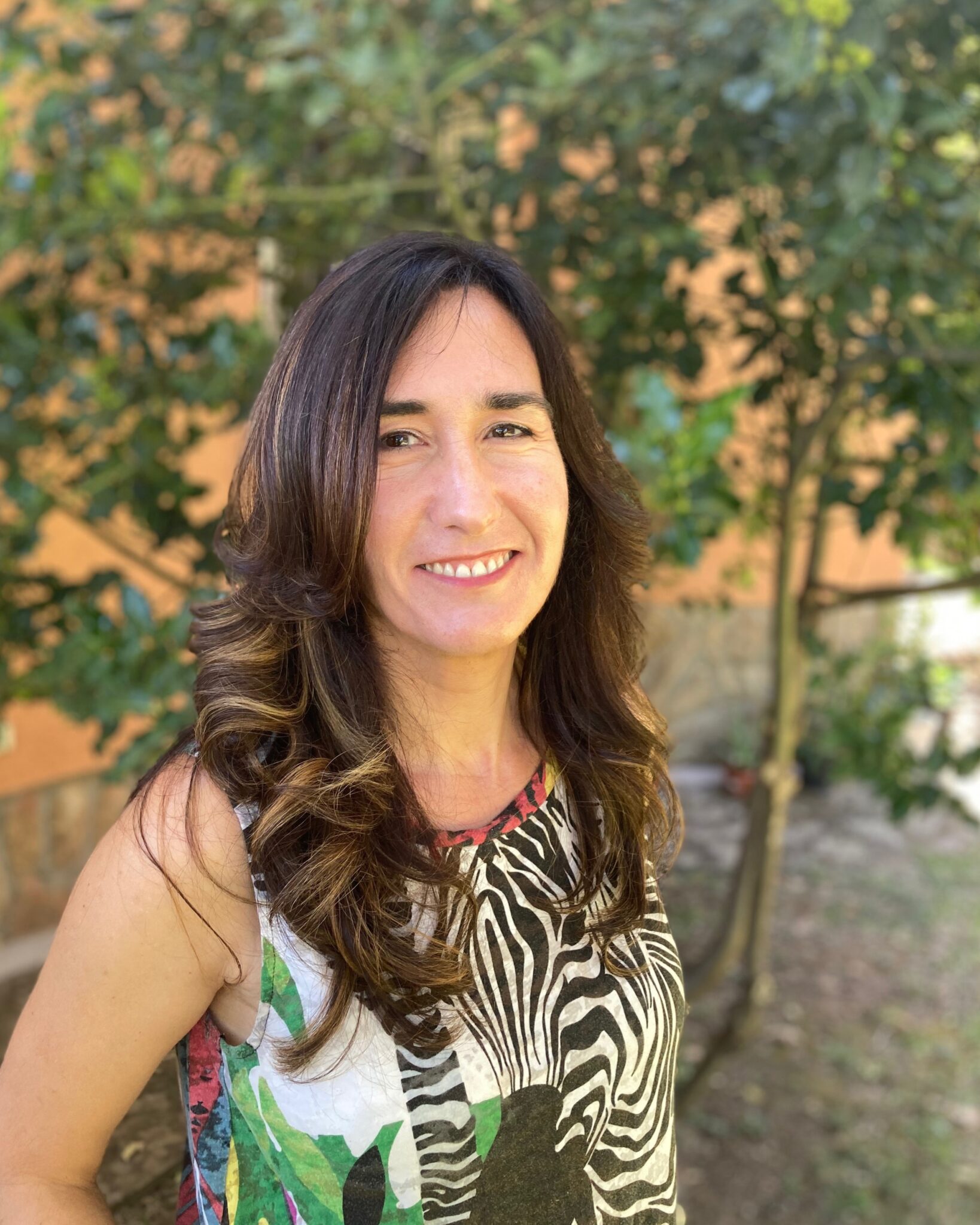
Sonia Diaz Martinez
Full Professor at the Department of Mechanical and Aerospace Engineering at the University of California, USA.