The list of accepted special sessions is available below. You can find more information on how to submit a Special Session Paper at the Paper Submission page.
Approved Special Sessions (in alphabetical order)
CODE: 765y4
In alignment with the IEEE CASE 2024 theme, “Automation 5.0: Automation Everywhere for Better and Smarter Living,” this special session delves into exploring cutting-edge methodologies for analysing and optimizing Automated Container Terminals (ACTs). ACTs are rapidly becoming the focal point of port development, driven by their notable efficiency, environmental sustainability, and safety features. However, the complexity inherent in these terminals, marked by large-scale operations, numerous equipment units, high-frequency concurrent tasks, intricate business interdependencies, and dynamic operational constraints, poses challenges for effective analysis, optimization, and evaluation. In recent years, with the rise of technologies such as the Internet of Things (IoT) and cloud computing, data within Terminal Operating Systems (TOS) has become increasingly precise and comprehensive. This data evolution enables us to enhance overall decision-making capabilities within the port by leveraging accurate and comprehensive information.
Therefore, recognizing the significance of addressing the challenges posed by the complexity of ACTs, our proposed special session aims to explore the latest advancements in their analysis and optimization, with a specific focus on integrating data for informed decision-making. This session will delve into state-of-the-art methodologies for analyzing and optimizing ACTs, emphasizing the harnessing of data integration to enhance decision-making capabilities and further refine the automation process.
Sub-topics
– Innovative approaches to optimizing the efficiency and sustainability of ACTs
– Integration of data analytics and artificial intelligence for enhanced decision-making in terminal operations
– Utilizing data, artificial intelligence, and digital twin technologies for informed decision-making in ACTs
– Application of large-scale language models to improve decision-making processes in terminal management
– Using advanced data analytics and probabilistic modelling to predict and manage operational uncertainties
– Application of edge computing technologies in ACTs for real-time data processing and decision-making
– Assessing the impact of 5G and other advanced wireless communication technologies on the operation and management of ACTs
– Examining the role of data integration in enhancing supply chain collaboration, visibility, and efficiency in connection with ACTs
– Exploring the use of data-driven approaches for optimizing energy consumption and implementing sustainable energy solutions in ACTs
– Leveraging data integration and analysis for risk assessment and mitigation strategies in ACTs, focusing on environmental risks, operational hazards, and cybersecurity threats
Organizers
– Wei Qin, Associate Professor, Shanghai Jiao Tong University. E-mail: wqin@sjtu.edu.cn
– Lu Zhen, Professor, Shanghai University. E-mail: lzhen@shu.edu.cn
– Runzhi Tan, Ph.D. candidate, Shanghai Jiao Tong University. E-mail: tanrunzhi@sjtu.edu.cn
– Zhanluo Zhang, Ph.D. candidate, Shanghai Jiao Tong University. E-mail: zhangzhanluo@sjtu.edu.cn
CODE: vwbk6
In the rapidly evolving landscape of urbanization, the challenges associated with transportation become increasingly complex. Intelligent Transportation Systems (ITS) have emerged as a crucial solution to address these challenges, leveraging cutting-edge technologies to optimize various facets of urban mobility. The importance of ITS extends beyond mere convenience; it plays a pivotal role in mitigating traffic congestion, enhancing energy efficiency, and ensuring the safety of commuters. In particular, the development of artificial intelligence technology, sensing, and communication technologies has brought unprecedented innovation to the transportation system. Autonomous driving, the Internet of Vehicles, smart logistics, and more have occurred. Central to the evolution of ITS is the development of novel control and optimization techniques that enhance their functionality, effectiveness, and safety.
This session serves to gather researchers and practitioners around the world to explore the advances in control and optimization methods of shaping the future of transportation in smart cities and to preview the next steps on the way.
Sub-topics
– Control of different transportation systems, including road traffic systems, railway systems, aircraft, etc.;
– Applications of artificial intelligence in transportation systems, including machine learning methods to traffic forecasting, computer vision, etc.;
– Logistic networks;
– Control of vehicles, including autonomous vehicles and platoons;
– Traffic management;
– Control of robotic systems for transportation applications
– Safety verification and performance analysis of transportation systems;
– Cybersecurity in transportation systems.
Organizers
– Yin Tong, Associate professor, Southwest Jiaotong University, China. E-mail: yintong@swjtu.edu.cn
– Silvia Siri, Associate Professor, University of Genova, Italy. E-mail: silvia.siri@unige.it
– Simona Sacone, Associate Professor, University of Genova, Italy. E-mail: simona.sacone@unige.it
CODE: 94dy6
Navigating the escalating demand for customized products while upholding stringent quality standards presents formidable challenges in the effective management of manufacturing systems. These challenges can be effectively met through the realization of autonomous manufacturing, minimizing human intervention. Autonomous manufacturing represents a transformative shift towards intelligent, self-governing production systems that leverage cutting-edge technologies to enhance efficiency, flexibility, and responsiveness within manufacturing processes. This necessitates the imperative adoption of advanced technologies such as reinforcement learning, deep learning, meta-learning, digital twin, and optimization methods. The urgency for autonomous manufacturing is particularly pronounced in industries like semiconductors, displays, batteries, and biopharmaceuticals, where production requirements encompass a myriad of complexities—ranging from time window constraints and limited buffers to sequence-dependent setup times, transport robots, and stringent quality control measures. To address these intricacies, this special session aims to bring together researchers, engineers, scientists, and managers actively involved in the research, development, and operation of manufacturing systems. The objective is to foster a collaborative effort to tackle the diverse challenges associated with modeling, scheduling, operation, and control for the advancement of autonomous manufacturing.
Sub-topics
– Reinforcement learning for autonomous manufacturing
– Meta-learning for scheduling of manufacturing systems
– Deep learning for quality control
– Optimization method for production efficiency
– Digital twin for autonomous manufacturing
– Real-time scheduling and control for autonomous manufacturing
– Modeling and control for autonomous manufacturing
– Optimization for automated manufacturing systems
Organizers
– Hyun-Jung Kim, Associate Professor, Department of Industrial & Systems Engineering, KAIST. E-mail: hyunjungkim@kaist.ac.kr
– Mengchu Zhou, Distinguished Professor, Department of Electrical and Computer Engineering, New Jersey Institute of Technology. E-mail: mengchu.zhou@njit.edu
CODE: 4n45i
The healthcare industry in many countries is facing significant challenges of rising costs, limited resources, increasing patient demand, and complex healthcare systems. Intelligent healthcare system integrating emerging technologies such as electronic health records, IoT devices, robotics and automation, AI, and telehealth and online healthcare might provide a promising solution. These technologies also raise challenges to healthcare management in terms of workflow adjustment, streamlining processes, data management, interoperability, ethics, privacy and security, medical quality, and cost and resource management that need to be addressed for effective implementation and utilization. In order for emerging technologies to better empower healthcare delivery systems, new methods and tools are being innovated to transform healthcare system by improving efficiency, decision-making, and patient care outcomes.
This special session aims to explore the advancements in intelligent healthcare management by researchers, practitioners, and policymakers from different disciplines. In this session, we welcome all contributions that relate to using the modeling and algorithmic approaches and software tools in operations management, medical decision, AI, data analytics, and policy making with application to addressing intelligent healthcare management problems.
Sub-topics
– Predictive analytics to forecast patient demands and resource requirements
– Healthcare system planning and design
– Operational planning in healthcare organizations
– Process optimization and design for healthcare service
– Intelligent algorithms for patient scheduling
– Healthcare quality management
– Healthcare supply chain management
– Health information systems and technology management
– Online healthcare management
– New methods or tools for Lean management for intelligent healthcare system
– Intelligent decision support systems for clinical decision-making
– AI-based novel approaches for intelligent healthcare management
– Human robot/intelligent-system collaboration for healthcare
– Healthcare policy making
– Healthcare innovation and entrepreneurship
Organizers
– Zhibin Jiang, Professor, Antai College of Economics and Management, Shanghai Jiao Tong University. E-mail: zbjiang@sjtu.edu.cn
– Xiaolan Xie, Professor, Mines Saint-Etienne, University Clermont Auvergne. E-mail: xie@emse.fr
– Liping Zhou, Associate Professor, Sino-US Global Logistics Institute, Shanghai Jiao Tong University. E-mail: zhoulp@sjtu.edu.cn
CODE: 1911f
Discrete event systems (DES) play a crucial role in modeling and analyzing dynamic systems across various domains, including manufacturing, transportation, healthcare, and telecommunications. With the rapid advancements in algorithm development and software tools, novel opportunities arise for enhancing the analysis, design, and application of DES. This special session aims to explore the latest advancements in software tools and cutting-edge applications of DES.
The session will explore state-of-the-art software tools that empower DES researchers and practitioners to model, simulate, analyze, and optimize complex dynamic systems. It will delve into the advancements in DES software, encompassing modeling languages, simulation platforms, optimization techniques, and integration frameworks. The session aims to provide insights into innovative approaches for system behavior analysis, performance evaluation, and decision support within the realm of DES. Moreover, this session will highlight the emergence of new DES applications that leverage software tools to address contemporary challenges in solving real-world problems.
Sub-topics
– Software tools of discrete-event systems
– New algorithms of discrete-event systems
– Algorithm implementation and testing
– Simulation platforms of discrete-event systems
– Experimental testbeds of discrete-event systems
– Case studies of discrete-event systems
– New applications of discrete-event systems
– Applications of discrete-event systems in cyber-physical systems
Organizers
– Kai Cai, Professor, Department of Core Informatics, Osaka Metropolitan University, E-mail: cai@omu.ac.jp
– Michel A. Reniers, Associate Professor, Department of Mechanical Engineering, Eindhoven University of Technology E-mail: m.a.reniers@tue.nl
– Martin Fabian, Professor, Department of Electrical Engineering, Chalmers University, Email: fabian@chalmers.se
CODE: 41pkv
Discrete event dynamic systems (DEDS) aim at studying the man-made systems driven by events, such as the systems of manufacturing, transportation, computer, communication, energy, robots, etc. The foundation of DEDS is built on mathematical models, such as Markov models, Petri net, automata, queueing models, etc. The decision and control of DEDS is fundamental to improve the operation efficiency of those man-made systems, which involves the optimization theory such as Markov decision process (MDP), optimal control, supervisory control, etc. Recently, the remarkable successes of AI attract intensive attention on the study of data-driven learning and optimization. One of the main research streams of AI is to handle the dynamic decision-making problem with reinforcement learning, whose mathematical foundation is MDP. Therefore, with these facts, the research development of DEDS theory encounters a crossroad, combining the techniques of AI and enabling the study of DEDS in a manner of data driven learning and optimization.
This special session aims to bring together the international scholars and industry practitioners to discuss the recent progress of DEDS in the background of big development of AI techniques, while focusing on the field of automation science and engineering. The potential topics include but are not limited to the development of DEDS theory such as Markov systems, Petri net, automata, the development of reinforcement learning & MDP decision theory, the AI enabled solution to dynamic games & multi-agent systems, and the application of above theories to solve engineering problems in the field of automation science and engineering.
Sub-topics
– Fundamental theory development of DEDS
– Controlled Markov systems
– Control in reinforcement learning
– Petri Nets for Automation Control- Formal Methods in Robotics and Automation
– New advancement in automata
– Security control and Supervisory control
– Control and management in queueing models
Organizers
– Qianchuan Zhao, Professor, Department of Automation, Tsinghua University, E-mail: zhaoqc@tsinghua.edu.cn
– Kai Cai, Professor, Department of Core Informatics, Osaka Metropolitan University, E-mail: cai@omu.ac.jp
– Xiang Yin, Associate Professor, Department of Automation, Shanghai Jiao Tong University, E-mail: yinxiang@sjtu.edu.cn
– Li Xia, Professor, School of Business, Sun Yat-Sen University, E-mail: xiali5@sysu.edu.cn
CODE: 25c9k
Remanufacturing, an integrated and complex industrial process, plays a critical role in international economy development and global ecosystem prosperity. Efficiency and sustainability are key components for the future of remanufacturing industries. Artificial intelligence (AI) and robotics are leading to deep workplace innovation, optimizing human-machine interactions, giving more importance to workers, and enabling efficient and sustainable industrial processes. However, the environmental goals can only be achieved by rethinking the disassembly processes in order to limit the ecological impact. Disassembly scheduling and optimization problems are the significant issues for an industrial remanufacturing process and affect its efficiency, energy consumption, production costs, safety, and stability. In recent years, the theory and technology of artificial intelligence and robotics have been developing vigorously. The applications of artificial intelligence technologies, like deep learning, machine learning, evolutionary computation, heuristic algorithms, cloud computing, internet of things, and big data analysis are gradually affecting all walks of life in the real world. Combining artificial intelligence and robotics with specific industrial remanufacturing processes to solve their intelligent disassembly scheduling and optimization problems is a high demand for industries and an important research topic in academia, which will be making remanufacturing lines as efficient and sustainable as possible including the design, optimization, improvement, and implementation of the collaborations between workers and machines. AI and robotics can help handle the complexity of these problems to find and implement solutions that increase efficiency and lower the impact of production on the environment. Related research can improve operation efficiency, enhance safety and stability, and reduce energy consumption and disassembly costs, which can further boost the level of intelligent remanufacturing in industrial remanufacturing processes.
Sub-topics
– Design, control, and optimization of disassembly systems
– Design, control, and optimization of assembly systems
– Robotics and human-robot interaction for disassembly tasks
– Digital twin design and development in remanufacturing
– Emission control and clean energy in remanufacturing
– End-of-life product recycling
– Petri nets, UML, queuing theory, model-checking techniques for remanufacturing systems
– Heuristic search algorithms
– Intelligent factory
– Real-time operation management, task allocation, and task scheduling
– AI and machine learning technologies in remanufacturing
– Smart sensing, control, and logistics management in remanufacturing
– Remanufacturing simulation, validation, and evaluation
– Workstation load balancing in manufacturing, assembly, and disassembly
Organizers
– Xiwang Guo, Associate Professor, IEEE Senior Member, Dept. of Information and Control Engineering, Liaoning Petrochemical University, China E-mail: x.w.guo@163.com
– Weitian Wang, Associate Professor, IEEE Senior Member, School of Computing, Montclair State University, USA, E-mail: wangw@montclair.edu
– Mengchu Zhou, Dist. Professor, IEEE Fellow, Dept. of Electrical and Computer Engineering, New Jersey Institute of Technology, USA, E-mail: zhou@njit.edu
CODE: 48gut
In the current era of intense global competition and rapid technological advances, manufacturers must frequently introduce new product variants. Each change in the product family requires the adaptation of manufacturing systems, and the frequent reconfiguration of production lines leads to sustainability challenges. Production resources are prematurely taken out of service, leading to scrapping or, at best, being sold for spare parts. In response to these challenges, the concepts of Industry 4.0 and Industry 5.0 have been introduced. Industry 4.0 represents significant improvements in production efficiency and flexibility through digitization and AI-driven technologies. Industry 5.0 takes these technological advances further, and it focuses on three fundamental principles: sustainability, human-centricity, and resilience. By emphasizing these pillars, Industry 5.0 seeks to address the environmental impact of manufacturing processes, improve the well-being of workers, and fortify systems against unforeseen disruptions. Despite its promising potential, the exploration of Industry 5.0 is still in its early stages, with limited research and a lack of systematic understanding. In this context, this session welcomes original theoretical approaches and new applications that improve sustainability, resilience, and human centricity during the design, maintenance, operation, and decommissioning of assembly lines.
Sub-topics
– Impact of Industry 4.0 and Industry 5.0 on production systems
– Assembly equipment circulation and its sustainability aspects
– Innovative ways to improve the sustainability of assembly lines
– Maintenance policy with second hand resources
– Worker and technician management in assembly lines
– Lifecycle management of production systems
– Resilience of production systems
– Human-centricity in production systems
– Integration of innovative and smart digital tools in production systems
– AI in assembly and assembly system planning
– Digital twins for assembly system modelling
– Middlewares and AAS in the context of second-hand assembly equipment
– Condition monitoring and predictive maintenance for assembly resources
Organizers
– Hamidreza Rezaei, Post-Doctoral Researcher, IMT Atlantique, Nantes, France. E-mail: hamidreza.rezaei@imt-atlantique.fr
– Simon Thevenin, Professor, IMT Atlantique, Nantes, France. E-mail: simon.thevenin@imt-atlantique.fr
– S. Ehsan Hashemi-Petroodi, Assistant Professor, KEDGE Business School, Bordeaux, France. E-mail: seyyed-ehsan.hashemi-petroodi@kedgebs.com
CODE: v6w54
With the accelerated grow of new IT today, control development is in a fast transition to the new stage of Automation 5.0. Internet of Things (IoT), big data, cloud computing, AI, robotics, augmented reality, virtual reality, and software-defined systems have provided the hardware/software infrastructure and content for Automation 5.0. The principle of Automation 5.0 is the virtual-real duality, i.e., intrinsically separating model representation from physical objects and making them independent. Over the past decade, ACP-based parallel systems approaches have been applied to many fields, from algorithm development for parallel learning, parallel games, parallel dynamic programming, prescriptive simulation, to real-world applications of parallel control, intelligence, management, and transportation. Knowledge automation, as industrial automation for modern industries, will be essential and critical for the success of parallel control and Automation 5.0. This special session aims to present the latest research and invite submissions for new works on cutting-edge methods and applications of Automation 5.0. We are soliciting original contributions that are not published or currently under consideration by any other journals/conferences.
Sub-topics
– Knowledge automation
– Parallel control for Automation 5.0
– Cyber-Physical Social Systems
– Virtual reality, Augmented Reality for Automation 5.0
– Robotics and control under Automation 5.0
– Human-centric automation with its applications
– Foundation model and foundation intelligence
– Automation 5.0 for smart manufacturing, smart mobility and smart society
Organizers
– Fei-Yue Wang, Professor, Chinese Academy of Sciences, E-mail: feiyue@ieee.org
– Chen Lv, Associate Professor, Nanyang Technological University, E-mail: lyuchen@ntu.edu.sg
– Hailong Huang, Assistant Professor, Hong Kong Polytechnic University, Email: hailong.huang@polyu.edu.hk
– Yutong Wang, Chinese Academy of Sciences, E-mail: yutong.wang@ia.ac.cn
CODE: yg995
Technology advancements, along with multiple significant issues such as lack of labour and general labour intensity in agriculture tasks, have sparked a rapid growth in agriculture and horticulture automation and robotics over the past 15 years. There are successful demonstrations in different aspects of agriculture and horticulture tasks such as crop and fruit harvesting, flower pollination and thinning, crop and fruit detection in different cycle phases and smart overall monitoring and management of weeds, crops, and fruits. However, it remains challenging for smart systems to achieve the dexterity, complexity, and speed of human motion in completing agriculture and horticulture tasks with full autonomy capability. In addressing these challenges, this special session aims to reflect on current advancements in automation and robotics technology in agriculture and horticulture that will eventually allow commercial adoption and application in farms, orchards, and greenhouses. The topics include advancements in perception, monitoring and grasping of agriculture and horticulture tasks, fundamental advancements, and applications in enabling full autonomy of ground or aerial vehicles (localization, navigation and mapping) in agriculture and horticulture conditions, systems of systems approach integration, human-robot collaborative work, safety and smart human-assisted tools for agriculture and horticulture tasks.
Sub-topics
– Precision agriculture
– Ground autonomous vehicles for agriculture and horticulture
– Aerial detection and applications for agriculture and horticulture
– Smart sensing applications for crops monitoring
– Smart manipulation and grasping control
– Human-robot collaboration and safety
– Design, modelling, and control of agricultural robots
– Automated harvesting systems
– Fruit detection and yield estimation
– Automated plant phenotyping systems
– Smart human-assisted tools
– Deep learning for perception and control
– Systems of systems approach
– Swarm robots for agriculture and horticulture
– Safety and reliability of autonomous robotics systems for agriculture and horticulture
Organizers
– Shen Hin Lim, Program Leader and Senior Lecturer of Mechatronics Engineering, School of Engineering, University of Waikato, New Zealand, hin.lim@waikato.ac.nz
– David A. Anisi, Associate Professor in Agriculture Robotics, Department of Mechanical engineering and technology management, Norwegian University of Life Science (NMBU), Norway, david.anisi@nmbu.no
– Ben McGuinness, Lecturer of Mechanical Engineering, School of Engineering, University of Waikato, New Zealand, ben.mcguinness@waikato.ac.nz
– Mike Duke, Dean of Engineering, School of Engineering, University of Waikato, New Zealand, mike.duke@waikato.ac.nz
– Antonio C. Leite, Associate Professor in Agriculture Robotics, Department of Mechanical engineering and technology management, Norwegian University of Life Science (NMBU), Norway, antonio.candea.leite@nmbu.no
– Weria Khaksar, Associate Professor in Agriculture Robotics, Department of Mechanical engineering and technology management, Norwegian University of Life Science (NMBU), Norway, weria.khaksar@nmbu.no
CODE: f89hf
Industrial web 3.0, as the next generation of industrial internet, aims to create a more intelligent and secure industrial network environment. Constructed on blockchain networks, it can support smart factories, predictive maintenance, and real-time monitoring of equipment and processes, with ensured data security and integrity, for many industries such as manufacturing, logistics, and supply chain management. Blockchain-empowered industrial web 3.0 represents a new decentralized paradigm, where users retain ownership of their generated industrial data. Such a paradigm shift necessitates a convergence of communication, networking, computation, and storage in a decentralized manner, with blockchain technology playing a pivotal role in establishing this trustworthy environment. However, to meet these requirements, blockchain technologies face significant challenges in scalability, trust establishment, data management, and application exploration. Therefore, this special session aims to bring together specialists in different fields of industrial engineering, blockchain systems, data science, artificial intelligence, and other engineering domains to address the foreseeable blockchain-empowered industrial web 3.0 paradigm characterized by high-level data security and transparency.
Sub-topics
– New blockchain architectures for industrial web 3.0
– Decentralized data management and analytics in industrial web 3.0
– Decentralized identifiers (DID) for industrial web 3.0
– Incentive and consensus schemes in industrial web 3.0
– Function-embedded smart contract for industrial web 3.0
– Blockchain with edge-cloud collaboration for industrial web 3.0
– Data security challenges and solutions in industrial web 3.0
– Systems and platforms in industrial web 3.0
Organizers
– Zhe Peng, Research Assistant Professor, The Hong Kong Polytechnic University. E-mail: jeffrey-zhe.peng@polyu.edu.hk
– Hong-Ning Dai, Associate Professor, Hong Kong Baptist University. E-mail: henrydai@comp.hkbu.edu.hk
– Ray Y. Zhong, Assistant Professor, The University of Hong Kong. E-mail: zhongzry@hku.hk
– Gangyan XU, Assistant Professor, The Hong Kong Polytechnic University. E-mail: gangyan.xu@polyu.edu.hk
– Ming Li, Research Assistant Professor, The Hong Kong Polytechnic University. E-mail: ming.li@polyu.edu.hk
– Zhiheng Zhao, Research Assistant Professor, The Hong Kong Polytechnic University. E-mail: zhiheng.zhao@polyu.edu.hk
– George Q. Huang, Chair Professor, The Hong Kong Polytechnic University. E-mail: gq.huang@polyu.edu.hk
CODE: 68e4e
Worldwide, modern manufacturing industries are undergoing a transition from technology-centric to value-centric development. These industries use innovative technologies to create and improve products, capitalizing on existing and future ICT infrastructure, including automation, computation, informatization, and digitalization. However, even with advancement in the development of data-rich and knowledge-intensive systems, these technologies predominantly center on knowledge extraction and pattern recognition. In contrast, cognitive-driven manufacturing places emphasis on the adoption of advanced concepts like self-learning, self-optimization, and self-healing (referred to as Self-X), thereby endowing manufacturing systems with the ability to perceive, comprehend, and learn autonomously. The development of cognitive-driven manufacturing facilitates a more profound understanding of market demands, optimization of production processes, and the innovation of products and services. This, in turn, propels the direction towards value-driven sustainability, enriched by heightened cognitive intelligence. Cognitive-driven intelligent technologies hold significant potential in various aspects, including human-machine/robot collaboration, innovative design, dynamic optimization, predictive maintenance, and decision support throughout different stages of the engineering lifecycle. However, the profitable and secure utilization of cognitive intelligence in manufacturing operations remains uncertain. Additionally, fundamental challenges persist, such as the alignment of data and knowledge architecture across multiple processes, entities, and stages. Hence, this special session aims to bring together specialists in smart/intelligent/advanced manufacturing, information and communication, artificial intelligence, cognitive computing, and other science and engineering domains to address these pressing yet long-standing industrial needs.
Sub-topics
– Advances in cognitive intelligence theories
– Fundamentals in cognitive manufacturing systems
– Cognitive computing for manufacturing
– Knowledge representation for cognitive intelligence
– Knowledge graph and graph-based reasoning
– Knowledge graph-based predictive maintenance
– Cognition in innovative product/system design
– Cognitive digital twin for manufacturing systems
– Cognitive human-robot collaboration systems
– Cognitive prognostics and health management
– Self-organizing manufacturing networks/systems
– Self-anomaly-detection in manufacturing processes
– Self-coordinating supply chains/networks
– Self-diagnosis of intelligent machines/products
– Cases, applications, and implementations in cognitive manufacturing systems
Organizers
– Tao Peng, Associate Professor, Zhejiang University, E-mail: tao_peng@zju.edu.cn
– Pai Zheng, Assistant Professor, The Hong Kong Polytechnic University, E-mail: pai.zheng@polyu.edu.hk
– Xinyu Li, Research Professor, Donghua University, E-mail: lixinyu@dhu.edu.cn
– Qingge Xiao, Assistant Professor, Shenzhen Institutes of Advanced Technology, E-mail: qg.xiao@siat.ac.cn
– Lihui Wang, Professor, KTH Royal Institute of Technology, E-mail: lihuiw@kth.se
– George Huang, Professor, The Hong Kong Polytechnic University, E-mail: gq.huang@polyu.edu.hk
– Weiming Shen, Professor, Huazhong University of Science and Technology, E-mail: wshen@ieee.org
CODE: 4inf3
During the Industry 5.0 revolution, robotics has become increasingly significant in advancing manufacturing automation and intelligence. Different from traditional industrial robots, which are fenced off from human workers on production lines, collaborative robots make a tremendous shift to coexist and collaborate with human workers in open environments for manufacturing tasks. This makes robots capable of democratizing manufacturing industries with dynamic customer demands, high flexibility, and low cost. In the human-robot collaborative advanced manufacturing, humans have unmatched problem-solving skills and develop creative and unique solutions compared to robots, which can provide physical assistance, free human partners from dangerous tasks, and lower the stress of human collaborators. Therefore, the cooperation and interaction between humans and robots for advanced manufacturing should create new potential opportunities and benefits to enhance safety, optimize production, boost efficiency, improve task quality, and increase worker productivity for industry sectors. In both academia and industries, new interdependent and cross-disciplinary research issues arise and need to be addressed to make human-robot teams more productive and ergonomic. This special session aims to bring researchers, engineers, scientists, and managers engaged in frontier research and technologies of robotics, automation, manufacturing, and cyber-physical systems to investigate and solve different open questions in the field of human-robot collaborative advanced manufacturing. Prospective authors are invited to share their state-of-the-art research findings to address the gaps in this area.
Sub-topics
– Learning from demonstrations for human-robot collaborative advanced manufacturing
– Modeling of human factors (e.g., trust, comfort) in human-robot interaction
– Human intention reasoning/prediction in human-robot collaboration
– AI-based approaches for advanced manufacturing in human-robot partnerships
– Multi-human-robot interaction for collaborative manufacturing tasks
– Advanced robot motion/action planning algorithms for human-robot collaboration
– Design and development of novel collaborative robots
– Ethical issues in human-robot interaction
– Virtual reality/augmented reality/extended reality for human-robot interaction
– Collaborative manufacturing task quality modeling and evaluation for human-robot teams
– Manufacturing task scheduling optimization in human-robot collaboration
– Development of smart sensory systems for human-robot collaborative advanced manufacturing
Organizers
– Weitian Wang, Associate Professor, IEEE Senior Member, School of Computing, Montclair State University, USA, E-mail: wangw@montclair.edu
– Xiwang Guo, Associate Professor, IEEE Senior Member, Dept. of Information and Control Engineering, Liaoning Petrochemical University, China, E-mail: x.w.guo@163.com
– Mengchu Zhou, Dist. Professor, IEEE Fellow, Dept. of Electrical and Computer Engineering, New Jersey Institute of Technology, USA, E-mail: zhou@njit.edu
CODE: 1614u
Small-batch customization/personalization greatly amplifies the complexity and uncertainty in manufacturing systems. On the one hand, the system complexity originates from the complexity of product structures, material types, and operational constraints caused by diversified demands, the complexity of dynamic interactions among heterogeneous human, machine, and material resources, as well as the spatiotemporal coupling constraints between production and logistics. On the other hand, uncertainties in various aspects such as demand, resources, and operations, will propagate and create chain reactions in the systems, causing chaos and damaging the overall system resilience.
Inspired by Cyber-Physical Systems (CPS), one the most promising technologies in Industry 4.0, Cyber-Physical Manufacturing Systems (CPMS) are characterized by the ubiquitous interconnection of multi-resource, transparency and traceability in operational processes, and accurate identification and location of uncertain disturbances. Through the integration of physical and cyber space, manufacturing operations are dramatically reshaped in CPMS, showing new opportunities to analyze and optimize manufacturing processes for small batch customization.
This special section aims to identify key technical/research challenges, investigate frontier research problems, and discuss novel methodologies for cyber-physical manufacturing in the mass customization/personalization era.
Sub-topics
– New models and methods for production planning, scheduling, and execution in CPMS
– Digitalization and machine intelligence in CPMS
– Production-intralogistics synchronization for CPMS
– Privacy and security computing for CPMS
– Spatio-temporal data analytics for CPMS
– Human-robot collaboration/interaction in CPMS
– Generative AI-aided operations in CPMS
– CPMS-enabled line balancing and material/part feeding
– CPMS-enabled resilient production and logistics
– CPMS-enabled system analytics and optimization
Organizers
– Ming Li, Research Assistant Professor, The Hong Kong Polytechnic University. E-mail: ming.li@polyu.edu.hk
– Mingxing Li, Lecturer, Jinan University. E-mail: limingxing@jnu.edu.cn
– Zhiheng Zhao, Research Assistant Professor, The Hong Kong Polytechnic University. E-mail: zhiheng.zhao@polyu.edu.hk
– Qiqi Chen, Postdoctoral Fellow, The Hong Kong Polytechnic University. E-mail: qiqi.chen@polyu.edu.hk
– George Q. Huang, Chair Professor, The Hong Kong Polytechnic University. E-mail: gq.huang@polyu.edu.hk
CODE: qsr78
Distributed industrial systems, e.g., Internet of Things (IoT), edge computing, and cloud computing, enable an environment that interconnects numerous heterogeneous physical devices such as mobile terminals, appliances, facilities, vehicles, factories, etc. to the Internet, thereby improving efficiency of various real-time ubiquitous applications such as manufacturing, urban computing, agriculture, and smart home. Currently, distributed industrial systems are usually implemented by following an architecture of the Cloud-centric Internet of Things where physical devices are interconnected to share computing, storage, and networking resources. However, distributed industrial systems currently face more big challenges in bandwidth, latency, energy, security, etc. In addition, applications suffer from tremendous data and require low-latency and local computation require that they are executed as close to data sources as possible. Given the growth in such application scenarios and recent advances in artificial intelligence algorithms, deep learning and inference at the edge and cloud are unfolding and increasing dramatically. Furthermore, recently emerging artificial intelligence algorithms significantly improve the performance of distributed industrial systems. Specifically, data-driven large-scale optimization algorithms are proposed recently to well handle high-dimensional compute-expensive problems in the design, implementation, and optimization of complex distributed industrial systems. The special session focuses on data-driven industrial intelligence for distributed systems, and aims to provide efficient scientific and engineering solutions to handle challenges and provide visions for future research and development of distributed industrial systems including smart factory, smart home, smart city, etc. In addition, state-of-the-art research findings on industrial IoTs, smart mobile devices, and big data analysis, are also highly related. In this way, this session can bring researchers and engineers in frontier research and technologies of deep learning, manufacturing, automation, and cyber-physical systems to investigate different open problems in the field of data-driven industrial intelligence for distributed systems.
Sub-topics
– Big data analysis for industrial systems
– Artificial intelligence-enabled IoT applications
– Collaborative training in edge and cloud
– Energy-efficient industrial Internet
– Edge computing architectures, systems, and applications
– Efficient edge-cloud data orchestration
– Cyber-security and privacy aspects of industrial systems
– Energy-efficient dynamic resource provisioning
– Big data-driven job prediction and scheduling
– Data-driven industrial automation
– Large-scale optimization for industrial Internet
– Industrial applications and case studies
Organizers
– Haitao Yuan, Associate Professor, IEEE Senior Member, School of Automation Science and Electrical Engineering, Beihang University, Beijing, China. E-mail: yuan@buaa.edu.cn
– Jing Bi, Professor, IEEE Senior Member, Faculty of Information Technology, Beijing University of Technology, Beijing, China. E-mail: bijing@bjut.edu.cn
– Jia Zhang, Professor, IEEE Senior Member, Department of Computer Science, Southern Methodist University, Dallas, TX 75206, USA. E-mail: jiazhang@smu.edu
– MengChu Zhou, Dist. Professor, IEEE Fellow, Dept. of Electrical and Computer Engineering, New Jersey Institute of Technology, USA. E-mail: zhou@njit.edu
CODE: 18jj9
One of the enabling technologies of the digital transition is Autonomous Systems, which are systems capable of automatically achieving a given goal without the intervention of a human operator. They are able to collect information about the environment in which they operate, process data from multiple sources, and determine and plan actions to be taken to optimize performance, ensuring the safety of people and the proper functioning of devices. There is no doubt that they will assume a crucial role in society.
The purpose of this Special Session is to propose new and innovative decision and control techniques for developing Autonomous Systems, thus enabling sustainable problem-solving in extremely diverse application fields, such as manufacturing processes, automotive, aerospace, defense, road and rail mobility, smart cities, home automation, energy networks, water networks, environmental monitoring, smart agriculture, logistics, telecommunications, biomedicine, green transition, etc.
Original articles from both scholars and practitioners are welcome, reporting on concepts and approaches for Autonomous Systems and addressing system and control engineering topics that include but are not limited to:
– Design and develop autonomous engineering systems, with applications to smart manufacturing, autonomous vehicles, smart grids, robotics, and many more.
– Develop smart control algorithms (e.g., AI-enabled control, data-driven control, vision-based control) for smart environments such as smart cities, autonomous vehicles and mobile robots, smart grids, sustainable mobility systems, smart buildings, and smart homes.
– Develop testing platforms for emerging techniques to advance engineering autonomous system applications (e.g., cyber-physical systems, digital twin techniques).
– Design automated and high-performance industrial systems, studying issues related to distributed control and supervision for systems composed of networks of sensors, actuators, and collaborative robots.
– Design and operation of autonomous systems to guarantee their reliability and security, ensuring their proper functioning even under uncertainty (robustness), – monitoring and predicting failures, ensuring that confidentiality and privacy requirements are not violated, countering both physical and cyberattacks, and designing secure processes in environments where automated and human systems interact.
Sub-topics
– Autonomous Systems for Automation
– Autonomous Systems for Smart Environments
– Autonomous Systems for Monitoring and Security
– Automation in manufacturing
– Networks of sensors and actuators
– Supervisory control
– Autonomous vehicles
– Cyberphysical systems
– Mobile robotics
– Networked control
– Smart grids and smart cities
– Cyberattacks and Cybersecurity
– Fault diagnosis and prognosis
– Human in the Loop.
Organizers
– Laura Giarrè, Full Professor, Università di Modena e Reggio Emilia – Italy. E-mail: laura.giarre@unimore.it
– Alberto Cavallo, Full Professor, Università degli Studi della Campania Luigi Vanvitelli – Italy. E-mail: alberto.cavallo@unicampania.it
– Federica Pascucci, Associate Professor, Università degli Studi Roma Tre – Italy. E-mail: federica.pascucci@uniroma3.it
– Raffaele Carli, Assistant Professor, Politecnico di Bari – Italy. E-mail: raffaele.carli@poliba.it
CODE: c9b7s
Resilience has been a central idea for development of complex systems such as the supply chain resilience management, power system resilience control and management, social-physics network management, etc. In the traditional, stability-based view of resilience, models for resilient network design and recovery are usually triggered by disruptions and performance deviations to return to some “normal” state. Nowadays, complex systems had to deal with crises characterized by small probability, huge destructiveness, uncertain contagiousness. The design, control, and evaluation of complex system faces challenges from multi-dimensional scene, multi-scale computing, and multi-spatiotemporal factors. It becomes clear that the innovation in modeling, optimization, and computational methods takes a deeper role in resilience management of complex systems.
With the development of big data technology, this special session aims to discuss the recent progress of design, control, and evaluation of resilient complex systems, especially in supply chain management, power system, and social-physics coupling systems. The potential topics include but are not limited to the evaluation of key and weak links in system networks under multiple crisis and scenarios, the prediction and evaluation methodology for system resilience, feedback optimization methods for network transient response characteristics, distributed computing framework for social-physical systems, and mechanism design for distributed social-physical systems.
Sub-topics
– Modeling the coupled dynamic propagation process of multiple types of risks
– Analysis of information system security thresholds based on network dynamics
– Prediction of long-short term crisis processes
– Optimization and control in improving system resilience
– Data-driven multi-scale complex system computing framework
– System recovery in the presence of disruptions
– Design of distributed mechanisms supporting complex systems
– Resilience management in coupling systems
Organizers
– Di Wang, Associate Professor, Department of Industrial Engineering and Management, School of Mechanical Engineering, Shanghai Jiao Tong University. E-mail: d.wang@sjtu.edu.cn
– Xiangyu Wu, Associate Professor, School of Electrical Engineering, Beijing Jiaotong University. E-mail: wuxiangyu@bjtu.edu.cn
– Lu Zhang, Associate Professor,School of Electrical Engineering,China Agricultural University. E-mail: zhanglu1@cau.edu.cn
– Jingsi Huang, Research Associate, Department of Industrial Engineering and Management, Peking University. E-mail: jingsi.huang@pku.edu.cn
– Feng Gao, Research Associate, Department of Industrial Engineering and Management, Peking University. E-mail: gaofeng@pku.edu.cn
CODE: 4fj1u
Digital twin, a concept integrating modeling and data analytics, has become increasingly more relevant to engineering system design, development and operation. Different from traditional model-based engineering, a Digital Twin includes a dynamic, virtual representation of a physical system or object managed through the lifecycle of the physical system to best support various engineering purposes. It continuously mirrors the real-world entity by incorporating real-time data and machine learning, employing multiple-level, multiple-fidelity models and simulations, and applying advanced reasoning and fusion for prediction and decision-making. Digital Twins can be applied in various engineering systems, including space vehicles, manufacturing, medical devices, and smart building and infrastructure. They are particularly useful for predicting performance, process control and optimization and maintaining assets like machines, energy networks or entire cities. This special session explores the frontier challenges and solutions associated with the design and application of digital twins for successfully engineering system development and operation.
Sub-topics
– The mathematical, statistical, and computational foundations of digital twins in engineering applications
– Key challenges in digital twin development for engineering applications
– The use of digital twins in the integration of the sensors and data in monitoring of engineering systems.
– Data analytics and AI-enabled algorithms and models for advanced DTs.
– Hybrid modeling methods, combining physical principles, machine learning algorithms and ontology.
– Model predictive control for digital twin.
– Digital twin and cyberphysical systems
– Multi-level, multi-fidelity digital twin for engineering application
– Technical innovations in software architectures, data pipeline and machine learning Ops for DT development.
– DT uncertainty quantification and correlations to the system’s performance
– Digital twin interoperability, composibility and compositionality
– DT lifecycle management
– Advanced methods and tools for applying DTs in engineering fields, including manufacturing, building, grid, and vehicle etc.
Organizers
– Yan Lu, Supervisory Industrial Engineer, National Institute of Standards and Technology. E-mail: yan.lu@nist.gov
– Feng Ju, Professor, Arizona State University. E-mail: fengju@asu.edu
– Min-Hsiung Hung, Professor, Department of Computer Science and Information Engineering Chinese Culture University, Taiwan, ROC. E-mail: hmx4@ulive.pccu.edu.tw
– Haw-Ching Yang, Professor, National Kaohsing University of Science and Technology. E-mail: hao@nkust.edu.tw
CODE: jb924
With the rapid applications of information and communication technologies (ICT), such as building information modelling (BIM), Internet of Things (IoT), radio frequency identification (RFID), and geographic information system (GIS), the construction industry is moving towards automation, industrialization, and cyber-physical integration. Digital twin is a comprehensive digital representation of the physical entity for cyber-physical convergence and synchronization, which contains the properties, conditions, and behaviors of the real-life objects using digital models and data. Digital twin can be used to establish the Cyber-Physical System (CPS) to facilitate decision making and daily operation in construction. The level of automation in modular construction is not as good as manufacturing automation. Instead, digitization is more appropriate than automation to facilitate manual construction operations. However, it is extremely challenging to deploy digitization devices in construction environments that are typically very large, unstructured, uncontrollable and highly dynamic in contrast with Industry 4.0 manufacturing factories.
This session provides an excellent forum for scientists, researchers, engineers, and industrial practitioners to meet and share experiences, theoretical knowledge, or case studies on the application of digital twins in smart construction. Authors are invited to submit full papers describing original research work associated with various aspects of digital twin application in smart construction, such as data processing, machine-learning model development, and application examples in MiC factories, construction logistics, prefabrication yards, and on-site construction.
Sub-topics
– Digital twin-enabled Modular Integrated Construction(MiC)
– Digital twin-enabled construction life cycle management
– Digital twin-enabled real-time locating system in smart construction
– Digital twin-enabled synchronization and decision-making in construction logistics
– Digital twin-enabled smart site safety system (SSSS)
– Digital twin-enabled construction automation and robotics
– Digital twin-enabled Sustainable construction engineering and management
– Digital twin and AI case studies in smart construction
Organizers
– Zhiheng Zhao, Research Assistant Professor, Department of Industrial and Systems Engineering, The Hong Kong Polytechnic University. E-mail: zhiheng.zhao@polyu.edu.hk
– Gangyan XU, Assistant Professor, Department of Aeronautical and Aviation Engineering, The Hong Kong Polytechnic University. E-mail: gangyan.xu@polyu.edu.hk
– Ray Y Zhong, Assistant Professor, Department of Industrial and Manufacturing Systems Engineering, The University of Hong Kong. E-mail: zhongzry@hku.hk
– Wei Pan, Professor, Department of Civil Engineering, The University of Hong Kong. E-mail: wpan@hku.hk
– Wei Wu, Research Assistant Professor, College of Mechanical and Vehicle Engineering, Chongqing University. E-mail: bravew@cqu.edu.cn
– Qiqi Chen, Postdoctoral Fellow, Department of Industrial and Systems Engineering, The Hong Kong Polytechnic University. E-mail: qi-qi.chen@polyu.edu.hk
– Ming Li, Research Assistant Professor, The Hong Kong Polytechnic University. E-mail: ming.li@polyu.edu.hk
– George Q. Huang, Chair Professor, Department of Industrial and Systems Engineering, The Hong Kong Polytechnic University. E-mail: gq.huang@polyu.edu.hk
CODE: a2962
Continuous efficiency improvement in industrial and non-industrial processes represents a crucial challenge in the current energy and digital transition and geopolitical situation. Efficiency can be associated to different processes, e.g., plants, machineries, and devices; efficiency can be referred to different points of view, e.g., energy and production. Ad hoc powerful drivers and tools are becoming crucial in this context; furthermore, suitable Key Performance Indicators (KPIs) are needed as metrics for evaluation and certification of efficiency.
Accurate process’ knowledge and investigation are required for the definition of significant and holistic drivers, tools, and KPIs associated to efficiency in industrial and non-industrial processes. In this context, accurate and significant data are needed; for this purpose, specific data selection, acquisition, storage and analysis procedures must be implemented. Drivers, tools, and KPIs, through data exploitation and process knowledge and investigation, can trigger different goals, such as targeting, evaluating, and certifying decarbonization, rational resource use, and plant performance. Drivers, tools and KPIs oriented to efficiency improvement can be implemented for industrial and non-industrial processes of different sectors such as hard-to-abate sectors, water resources systems, and buildings.
In this field, the impact of automation science and engineering is growing significantly. The fields of application are increasing also thanks to cross-fertilization between different areas: well-established technologies in a defined area can be extended and customized for other areas. Automation science and engineering systems could be located at different levels of the automation hierarchy; tailored drivers and tools can be detected and developed, while profiting from KPIs in order to evaluate and certify performance and benefits through an objective and unbiased paradigm.
The present Special Session aims to collect contributions related to drivers and tools for efficiency improvement in industrial and non-industrial processes with a focus on the impact of automation science and engineering in this field. The Special Session would embrace emerging technologies and best practices. The Special Session will take into consideration research works on simulations in virtual environments and field applications. In addition, contributions on methodologies aimed at bridging the gap between simulations and field application are welcome, together with works on the transition from research to large-scale deployment.
Sub-topics
– Energy management system
– Decision Support System
– Expert system
– Advanced Process Control
– Real-time optimization
– Model Predictive Control
– Artificial Intelligence
– Discrete Event Systems
– Big Data
– Design
– Retrofit
– Operation
– Maintenance
Organizers
– Zanoli Silvia Maria, Università Politecnica delle Marche. E-mail: s.zanoli@univpm.it
– Pepe Crescenzo, Università Politecnica delle Marche. E-mail: c.pepe@univpm.it
CODE: 1v848
Manufacturing is characterized by capital/labor-intensive, the short product life cycle, rapid technology migration, long production lead-time, and complex production networks. These characteristics bring more challenges and difficulties to the manufacturing management. This session focuses on how the data science or machine learning techniques support problem-solving and enhance the core competence in manufacturing industry. The special session focuses on data science and engineering in the broad area of manufacturing. Theoretical research or empirical study are all welcome. The topics in this session include defect classification, maintenance scheduling, predictive maintenance, and process parameter optimization, etc.This session would like to provide a platform that offers opportunities to discuss, debate, and exchange ideas, in particular, in a world-side view of manufacturing system. We invite all the researchers, scholars, and graduates when they would like to develop the mathematical/empirical models and benefit the automation and data science field.
Sub-topics
– Smart manufacturing and factory automation
– Agent-based collaborative automation systems
– Automated fault detection, diagnostics, and prognostics
– Big data, data mining, and machine learning
– Cyber physical production systems and industry 4.0
– Modeling, simulation, and optimization of automation systems
– Capacity planning and scheduling
– Smart logistics and supply chains
– Sustainability and green manufacturing
– Digital Twins and Simulation Models
– Process Mining for Manufacturing
Organizers
– Chia-Yen Lee, Professor, National Taiwan University, Taiwan, E-mail: chiayenlee@ntu.edu.tw
– Chia-Yu Hsu, Professor, National Taiwan University of Science and Technology, Taiwan, E-mail: cyhsu@ntust.edu.tw
– Shu-Kai S. Fan, Professor, Shu-Kai Fan, National Taipei University of Technology, E-mail: morrisfan@ntut.edu.tw
– Feng Ju, Associate Professor, Feng Ju, Arizona State University, US, E-mail: fengju@asu.edu
– Jakey Blue, Associate Professor, Jakey Blue, National Taiwan University, Taiwan, E-mail: jakeyblue@ntu.edu.tw
– Young Jae Jang, Professor, KAIST, South Korea, E-mail: yjang@kaist.edu
– Anders Skoogh, Professor, Chalmers University of Technology, Sweden, E-mail: anders.skoogh@chalmers.se
– Giovanni Lugaresi, Assistant Professor, KU Leuven, Belgium, E-mail: giovanni.lugaresi@kuleuven.be
CODE: 36e2s
Energy Communities (ECs) are considered the future of smart grids, as they efficiently integrate distributed energy resources by promoting end-user self-consumption. ECs result from a contemporary and constantly evolving regulatory framework that authorizes private and public entities to establish economic contracts to share distributed energy resources. An EC is defined by end-users in a nearby location with electrical and/or thermal energy needs and access to individual or shared renewable energy sources and energy storage technologies. These communities have the potential to provide benefits from many points of view. Reducing transmission and distribution power losses can determine economic and environmental benefits. As they propose as a community framework, they also provide a social benefit by increasing end-user participation.
Of course, providing the best possible management of these entities requires a synergistic combination of control and optimization algorithms combined with information and communication technology (ICT).
A central aspect of this is the relationship between the EC and its neighbours. Effective coordination among entities is crucial for delivering network services within the EC framework. These entities’ flexibility can be useful for service providers, including aggregators. Such policies can be highly effective in optimizing grid management. The optimal coordination of ECs is a crucial topic in the evolution of the energy market.
With this special session, substantial contributions to the management and control of ECs and their optimal design are expected. Specifically, both approaches from an internal and external EC perspectives (multiple ECs to be managed) will be considered.
Sub-topics
– Optimal design of Energy Communities
– Distributed control and optimization for Energy Communities
– Machine learning-based control and optimization for Energy Communities
– Optimal control applied to Energy Communities
– Peer-to-peer energy sharing of individual/shared resources in Energy Communities
– Control, optimization, and communication interconnected Energy Communities.
– Cyber-physical security and cyber-physical human systems of large-scale Energy Communities
– Application to real Energy Communities
– Application to interconnected Energy Communities
Organizers
– Luca Parodi, Post-Doc, University of Genoa, E-mail: luca.parodi@edu.unige.it
– Virginia Casella, PhD Student, University of Genoa, E-mail: virginia.casella@edu.unige.it
– Michela Robba, Assoc Professor, University of Genoa, E-mail: michela.robba@unige.it
– Giulio Ferro, Asst Professor, University of Genoa, E-mail: giulio.ferro@unige.it
– Raffaele Carli, Asst Professor, Polytechnic of Bari, E-mail: raffaele.carli@poliba.it
– Paolo Scarabaggio, Asst Professor, Polytechnic of Bari, E-mail: paolo.scarabaggio@poliba.it
– Luigi Glielmo, Full Professor, University of Napoli Federico II, E-mail: luigi.glielmo@unina.it
– Giuseppe Notarstefano, Full Professor, University of Bologna, E-mail: giuseppe.notarstefano@unibo.it
CODE: 6ptpf
In line with the human-centric concerns of Industry 5.0, modern factories are striving for an ever-higher degree of flexible and resilient production, as conventional automation approach has reached its bottleneck considering mass personalization with increasing complicatedness and complexity. To achieve it, human-robot collaboration (HRC) becomes a prevailing strategy, which combines high accuracy, strength, and repeatability of industrial robots with high flexibility and adaptability of human operators to realise optimal overall productivity. Cutting-edge technologies, including robot learning and control, cognitive computing, mixed reality/metaverse, industrial IoT, and advanced data analytics create the potentials to bridge the gap of knowledge distilling and information sharing between onsite operators, robots and the manufacturing system with mutual cognitions. Therefore, this special session aims to bring together specialists in different fields of manufacturing systems, robotics, artificial intelligence, and other engineering domains to address the foreseeable HRC-empowered human-centric smart manufacturing paradigm characterized with high-level teamwork skills.
Sub-topics
– Human-robot collaborative disassembly
– Predictable human-robot-task execution loop
– Cognitive human-robot collaboration systems
– MR/Metaverse-assisted human-robot collaboration
– Self-organizing multiple human-robot collaboration
– Intuitive safety concerns in human-robot collaboration
– Multi-modal Intelligence for human-robot collaboration
– Adaptive motion planning in human-robot collaboration
– Human intention prediction in human-robot collaboration
– Semantic knowledge representation for human-robot collaboration
– Human-robot collaboration cases, systems, and implementations in manufacturing
Organizers
– Pai Zheng, Assistant Professor, The Hong Kong Polytechnic University, E-mail: pai.zheng@polyu.edu.hk
– Jinsong Bao, Professor, Donghua University, E-mail: bao@dhu.edu.cn
– Tao Peng, Associate Professor, Zhejiang University, E-mail: tao_peng@zju.edu.cn
– Shufei Li, Postdoctoral Fellow, The Hong Kong Polytechnic University, E-mail: shu-fei.li@polyu.edu.hk
– Wenjun Xu, Professor, Wuhan University of Technology, E-mail: xuwenjun@whut.edu.cn
– Xi Vincent Wang, Associate Professor, KTH Royal Institute of Technology, E-mail:wangxi@kth.se
– George Huang, Professor, The Hong Kong Polytechnic University, E-mail: gqhuang@hku.hk
– Lihui Wang, Professor, KTH Royal Institute of Technology, E-mail: lihuiw@kth.se
– Duc Truong Pham, Professor, University of Birmingham, E-mail: d.t.pham@bham.ac.uk
CODE: bq993
Complex networks make an enticing research topic that has been increasingly attracting researchers from control systems and various other domains over the last two decades. The rapid evolution and diffusion of ICT is leading to the implementation of complex systems that rely on communication, computation, and control techniques for their proper functioning.
Real-time feedback control systems integrate sensors, controllers, actuators, and communication networks. In this perspective, the control of complex systems implies that the network used for the communication of control actions is general purpose and used for various simultaneous applications. Moreover, the functionalities of the control level must be diversified from the pure automatic control. Consequently, it becomes challenging to ensure real-time communications in the whole system and to guarantee high performance, reliability, resilience, and stability.
Identification, control, and optimization of complex systems also involves constructing accurate models that represent the system’s behaviour. Artificial Intelligence (AI) and Machine Learning (ML) algorithms prove valuable in learning patterns from data, generating models capable of predicting system responses and devising effective strategies for controlling dynamic environments. Edge AI enables the implementation of ML models directly on the devices collecting data from the complex system, allowing for rapid processing and analysis of data, reducing latency and enabling quicker responses.
The objective of this Special Session is to collect recent research and development efforts contributing to advances in identification and control of complex systems, including state-of-the-art reviews and perspectives on future advances and applications.
Sub-topics
– Complex Systems
– Control Theory
– Autonomous Systems
– Discrete Event Systems
– Event-Trigger and Self-Trigger Control
– Digital Control
– Robotics and Automation
– Factory 4.0
– Information and Communication Technologies
– Learning Methods
– Big Data and Machine Learning
– Edge Artificial Intelligence
Organizers
– Nicola Epicoco, Assistant Professor, Libera Università Mediterranea, Bari (Italy). E-mail: epicoco@lum.it
– Mario Di Ferdinando, Assistant Professor, University of L’Aquila (Italy). E-mail: mario.diferdinando@univaq.it
– Giuseppe Loseto, Associate Professor, Libera Università Mediterranea, Bari (Italy). E-mail: loseto@lum.it
CODE: ypg7k
Foundation models, characterized by extensive parameters and large-scale training data, stand as strong tools equipped with broad knowledge gleaned from training data. These foundation models showcase prowess in reasoning, abstraction, and interaction in common fields. Despite their versatile capabilities, existing foundation models face challenges in specific domains such as smart manufacturing, primarily due to the absence of domain-specific knowledge.
In the domain of smart manufacturing, contemporary factories aspire to elevate flexibility, quality, and intelligence in their production processes. Conventional automation methods, constrained in interaction, flexibility, and decision-making, necessitate transformative solutions. Here, foundation models emerge as valuable solutions with their substantial advancements.
This special session is dedicated to Industrial Foundation Models (IFMs), with the aim of enriching foundation models with more domain-specific knowledge tailored for industrial scenarios. The primary objective is to navigate the intricacies of IFM development, addressing challenges related to processing extensive industrial data and formulating innovative training schemes tailored to the unique demands of industrial scenarios. These training schemes may include training IFMs from scratch, fine-tuning existing foundation models for specific industrial domains, new use of foundation models in the industry, and more. With a focus on smart manufacturing, this session explores the potential of IFMs in automating processes, enhancing capabilities for both humans and machines. Furthermore, it delves into the transformative possibilities of IFMs, envisioning their pivotal role in reshaping specific tasks within smart manufacturing, including product quality monitoring, prognostics and health management, and operations management, among others.
In essence, this special session aims to act as a catalyst for the development of IFMs and their applications in smart manufacturing, fostering advancements and efficiencies across diverse applications.
Sub-topics
– Training Schemes for IFMs
– Enhancing Product Quality Monitoring (online/offline) with IFMs
– Prognostics and Health Management using IFMs
– Optimizing Operations Management with IFMs
– Human-Machine Interaction Enhanced by IFMs
– Process Parameter Optimization using IFMs
– Design Optimization through IFMs
– Worker Training using IFMs
– Integrating IFMs with IoT for Smart Manufacturing
– IFMs for Supply Chain Optimization
– AI Ethics in Industrial Settings
– New applications of foundation models in industry.
Organizers
– Weiming Shen, School of Mechanical Science & Engineering, Huazhong University of Science and Technology, China, wshen@ieee.org
– Mengchu Zhou, Department of Electrical and Computer Engineering, New Jersey Institute of Technology, USA, mengchu.zhou@njit.edu
– Giacomo Boracchi, Department of Electronics, Information and Bioengineering, Polytechnic of Milan, Italy, giacomo.boracchi@polimi.it
– Kai Xu, School of Computer Science, National University of Defense Technology, China, kevin.kai.xu@gmail.com
– Yunkang Cao, School of Mechanical Science & Engineering, Huazhong University of Science and Technology, cyk_hust@hust.edu.cn
CODE: ef139
Industrial Internet of Things (IIoT) is characterized by time-series data, especially time-series databases such as Apache IoTDB, which are widely applied to various industrial scenarios covering air, space, ground, and ocean. Massive industrial time-series data can be efficiently collected, stored, and queried. In this context, industrial time series data-driven intelligent decision-making technologies have become a hot topic to achieve quality improvement, efficiency enhancement, energy conservation, and cost reduction of industrial production. However, complex industrial systems with unclear mechanisms, large amounts of data but limited valuable data, and the coexistence of structured and unstructured multimodal data significantly challenge the most advanced time-series data analysis methods, including Transformer. Fortunately, more advanced and interpretable models such as complex networks, causal analysis, and improved Transformers have broken through, and the large language model ChatGPT has gradually emerged with intelligence, and data science and AI for Research (AI4R) have become the fourth and fifth paradigms of modern scientific research, bringing dawn to addressing these challenges.
Therefore, this special session focuses on cutting-edge theories, models, and algorithms to reveal the mechanisms in complex industrial scenarios from industrial time series data and then integrate industrial mechanisms to achieve effective intelligent decision-making, bridging AI4R and engineering applications.
Sub-topics
– Advanced theory, methods, and industrial applications of time series data analysis
– Integrate data, AI, and digital twins for industrial decision-making
– Complex networks and causal inference for revealing the mechanisms of industrial systems
– Condition monitoring, anomaly diagnosis, and quality improvement through machine learning and deep learning
– Data science and AI4R for industrial decision-making
– Large language models for industrial decision-making
– Cross-research of data science, graph theory, and AI4R in mechanical engineering, control science, industrial engineering, and biomanufacturing
– Innovative cases in aerospace, marine engineering, micro nanomanufacturing, and industrial robotics
Organizers
– Yan-Ning Sun, Lecturer, Shanghai University, E-mail: sunyanning@shu.edu.cn
– Lilan Liu, Professor, Shanghai University, E-mail: lancy@shu.edu.cn
– Chen Wang, Associate Professor, Tsinghua University, E-mail: wang_chen@tsinghua.edu.cn
– Zenggui Gao, Associate Professor, Shanghai University, E-mail: gaozg@shu.edu.cn
– Wei Qin, Associate Professor, Shanghai Jiao Tong University, E-mail: wqin@sjtu.edu.cn
– Jianmin Wang, Professor, Tsinghua University, E-mail: jimwang@tsinghua.edu.cn
CODE: 1p9dy
To meet the rising food demand of a world population expected to reach 9.8 billion by 2050 while ensuring environmental sustainability, crop production must be improved by introducing new technologies and To meet the rising food demand of a world population expected to reach 9.8 billion by 2050 while ensuring environmental sustainability, crop production must be improved by introducing new technologies and artificial intelligence to accelerate the current transition to the Agriculture 4.0 paradigm. Agricultural robotics has advanced impressively, with numerous applications demonstrated. These include automated fruit picking, pruning, crop phenotyping and monitoring, weed management, selective pesticide and fertilizer spraying, and more.
To further enhance the capabilities and autonomy of farmer robots in demanding agricultural settings, both in open fields and greenhouse conditions, new challenges, such as motion planning and control, manipulation, learning, perception, and locomotion, need to be addressed. The interdisciplinary nature of agricultural robotics, which encompasses both robotics and natural sciences, is a recurring theme.
The purpose of this Special Session is to propose new and innovative techniques in in-field digital technology, agricultural robotics, and artificial intelligence. We welcome original articles from both scholars and practitioners reporting on concepts and approaches for enabling robotic systems in agriculture. The focus will be on strengthening the link between robotics and plant sciences in order to solve real-world problems. Papers that combine design and analysis and investigate disruptive technologies such as artificial intelligence and the Internet of Things, as well as the study of innovative locomotion systems, including passive/active articulated suspension systems for wheeled robots, articulated tracked vehicles, legged robots, and hybrid, reconfigurable, and bio-inspired architectures, are of particular interest.
Sub-topics
– Sensor and robot networks in agriculture
– Multisensor and data fusion
– Machine learning and deep learning from sensor data in agriculture
– Environment mapping and classification
– Robot localization, control and navigation
– Innovative locomotion systems for farmer robots
– In-field phenotyping sensors and vehicles
– Aerial and ground robotic platforms for agricultural applications
– Safe human–robot interaction in agricultural applications
– Sensors and vehicles for in-field pest detection and management
– Harvesting automation
– Cooperative agricultural robotics
– Safety and health in agriculture
– Food security
Organizers
– Giulio Reina, Full Professor, Polytechnic University of Bari. E-mail: giulio.reina@poliba.it
– Annalisa Milella, Senior Researcher, National Research Council of Italy. E-mail: annalisa.milella@cnr.it
– Yael Nemirovsky, Professor Emeritus, IEEE Life Fellow, Technion – Israel Institute of Technology, E-mail: nemirov@ee.technion.ac.il
– Andrea Gasparri, Full Professor, Università degli Studi “Roma Tre”, E-mail: gasparri@dia.uniroma3.it
Endorsement by the IEEE RAS Agricultural Robotics and Automation (AgRA) technical committee (http://ieeeagra.com/)
CODE: q392m
This special session aims to explore recent advancements and challenges in the integration of robotics and automation into healthcare practices. This session seeks to address the latest developments in diagnostic and therapeutic applications, minimally invasive surgery, rehabilitation, daily-life assistance, and efficient management of healthcare procedures. It provides a platform for interdisciplinary discussions among researchers.
The session is justified by the need to foster collaboration and share insights in the rapidly evolving field of robotics and automation applied to healthcare. Robotics and automation can significantly enhance patient care by providing precise and efficient medical procedures, reducing human error and complications, and enabling faster recovery times for patients. In cases where the availability of healthcare professionals is short, robotics and automation technologies can optimize workforce utilization, through e.g., telemedicine, faster surgical procedures, shorter hospital stays. This session intends to provide a platform to discuss advancements, share latest findings, and address ethical and practical considerations associated with automation and robotics in healthcare.
Participants will gain insights into research on robots operating in the human body, surgical robotics, rehabilitation robotics, daily-life and personal assistance devices, wearable technologies, and cognitive robotics. The session aims to inspire collaborative research initiatives and to accelerate the advancements of healthcare robotics.
Sub-topics
– Robotic devices for surgery (minimally invasive surgery)
– Robotic devices and interfaces for applications in the rehabilitation of people affected by movement disorders
– Robotic devices and interfaces for daily-life assistance of people affected by movement disorders
– Wearable technologies for medical applications and neuro-rehabilitation treatments
– Medical devices and cognitive robotics for human-robot interactions at cognitive and physical levels
– Robotic avatars and teleoperation for advanced remote health monitoring and assistance
– Automation and control technologies for assistance and support of healthcare workers in hospitals
– Advanced control methods for robots in medical and healthcare applications
Organizers
– Sanalitro Dario, Assistant Professor, University of Catania. E-mail: dario.sanalitro@unict.it
– Ferrentino Enrico, Assistant Professor, University of Salerno, E-mail: eferrentino@unisa.it
– Roveda Loris, Senior Researcher, IDSIA-SUPSI/USI, E-mail: loris.roveda@supsi.ch
– Palleschi Alessandro, Assistant Professor, University of Pisa, E-mail: alessandro.palleschi@phd.unipi.it
CODE: v326x
The mobile equipment could include but is not limited to agricultural machinery, mineral machinery, building machinery, and airfield equipment. Compared to manufacturing equipment, they could move directly or be transported to workplaces; compared to vehicles, they should finish some particular tasks while traveling. While the concept of mobile equipment has the potential to drive systems more efficient and productive, there are new Maintainence-Repair-Operation (MRO) challenges. The complex connectivity among variable working requirements, limited resources, complex working conditions, and different working places require novel methodologies to analyze, manage, and apply such equipment. Hence, this special session aims to motivate original, rigorous, and relevant research focusing on Intelligent MRO for mobile equipment. Another goal is to attempt to establish a strong researcher network in this emerging domain and stimulate cross-disciplinary research and discussions with researchers from various disciplines.
Sub-topics
– Mobile equipment scheduling and routing planning
– Maintenance network design for mobile equipment
– Maintenance resources allocation for mobile equipment
– Real time monitoring technology for mobile equipment
– IoT-based mobile equipment management system
– Data security and privacy in MRO for mobile equipment
– Intelligent mobile equipment under complex conditions
– AI-enabled Prediction of mobile equipment for MRO
Organizers
– Yi-Jia Wang, Distinguished Professor, Northeast Agricultural University. E-mail: yijiaw@connect.hku.hk
– Guizhen Yu, Professor, Beihang University. E-mail: yugz@buaa.edu.cn
– Yaoguang Hu, Professor, Beijing Institute of Technology. E-mail: hyg@bit.edu.cn
– Gangyan Xu, Assistant Professor, The Hong Kong Polytechnic University. Email: gangyan.xu@polyu.edu.hk
– Songyuan Zhang, Associate Professor, Harbin Institute of Technology. E-mail: zhangsy@hit.edu.cn
– George Q. Huang, Chair Professor, The Hong Kong Polytechnic University. E-mail: gq.huang@polyu.edu.hk
CODE: m8cq2
Operational optimization of complex systems is a multifaceted challenge that necessitates the infusion of intelligent approaches, like machine learning, reinforcement learning, evolutionary computation, and heuristic algorithms. Combining artificial intelligence technology with the characteristics of a specific complex system to solve its intelligent operation, maintenance, and scheduling problems is a hot research field of academia and an important demand of practical application. Related research can improve operation efficiency, ensure safety and stability, reduce energy consumption, and save production costs.
While existing research has made notable strides in tackling these complex issues, numerous related problems persistently remain open, necessitating further investigation and exploration. The research gaps of these challenges present exciting opportunities for researchers and practitioners to delve deeper into uncharted territories and contributing to the ongoing discourse on intelligent system optimization.
This special session aims to delve into the intricate realm of intelligent operation, maintenance, and scheduling within complex systems and provide a platform to exchange research results, technical trends, and practical experience related to fault diagnosis, process control, operation research, applied mathematics, and management science. Besides, this session is expected to broaden the intelligent optimization community and promote the application of artificial intelligence in practical complex systems.
Sub-topics
– Knowledge, mechanism and data-driven decision making
– Predictive-reactive scheduling in dynamic scenarios
– Digital twin and optimization for industrial equipment
– Multi-objective optimization and decision making
– Fault detection, performance prediction, and preventive maintenance
– Human-machine collaboration in multi-agent systems
– Optimal allocation of resources and energy in complex systems
– Cloud-edge-end collaboration for integrated operation and maintenance optimization
– Interdisciplinary collaboration for system optimization
Organizers
– Ziyan Zhao, Assistant professor, Northeastern University. E-mail: zhaoziyan@mail.neu.edu.cn
– Shixin Liu, Professor, IEEE Senior Member, Northeastern University. E-mail:sxliu@mail.neu.edu.cn
– Kaixiang Peng, Professor, IEEE Member, University of Science and Technology Beijing. E-mail:kaixiang@ustb.edu.cn
– MengChu Zhou, Distinguished Professor, IEEE Fellow, New Jersey Institute of Technology. E-mail: zhou@njit.edu
– Kai Zhang, Associate Professor, University of Science and Technology Beijing. E-mail:kaizhang@ustb.edu.cn
– Shuwei Zhu, Assistant professor, Jiangnan University. E-mail: zhushuwei@jiangnan.edu.cn
CODE: f4293
Learning assisted intelligent optimization and scheduling is a hot topic in recent years. This session focuses on the theory, algorithms and applications of learning assisted intelligent optimization and scheduling. It aims specifically at the most recent developments of swarm intelligence, evolutionary algorithms, meta-heuristics, ensemble with machine learning algorithms, and applications for various complex scheduling and optimization problems in engineering and service fields. Prospective authors are invited to share their academic results and practical experiences to deal with these challenging issues in this area.
Sub-topics
– Learning assisted multi-objective, multi-task, and multi-constraint optimization and scheduling
– Learning assisted large-scale global optimization and scheduling
– Ensemble swarm and evolutionary algorithms with machine learning algorithms
– Learning-based meta-heuristics
– Swarm intelligence and evolutionary algorithms for optimization and scheduling
– Clustering, classification and prediction based intelligent optimization and scheduling
– Deep reinforcement learning based optimization and scheduling
– New real-world and innovative applications of ensemble intelligent optimization with machine learning algorithms
Organizers
– Kaizhou Gao, Assistant Professor, Macau Institute of Systems Engineering,
– Macau University of Science and Technology, Macau, E-mail: kzgao@must.edu.mo
– Yan Qiao, Associate Professor, Macau Institute of Systems Engineering,
– Macau University of Science and Technology, Macau, E-mail: yqiao@must.edu.mo
– Yaping Fu, Associate Professor, School of Business, Qingdao University, Qingdao, E-mail: fuyaping@qdu.edu.cn
CODE: 5r889
Machine learning has demonstrated strong capability in addressing challenging decision making tasks in various fields in automation, such as autonomous driving, energy management, and manufacturing scheduling etc. The purpose of this special session is to focus on the recent development in machine learning for optimization in automation. This includes but does not limit to modeling, solving, and analyzing the optimization problem, both for parameter optimization and for dynamic decision making problems. In particular, we welcome submissions that discuss how machine learning helps to address optimization problems in general such as in linear and nonlinear mixed integer programming, or Markov decision processes. Advances in this direction would benefit applications across multiple domains. Specific applications such as in autonomous driving, energy management, and manufacturing, among others, are also welcome. The special structural property in these systems might provide insight on how machine learning techniques might further advance optimization algorithms in these domains. The projected outcome is to provide a state-of-art review of the latest advances in machine learning for optimization in automation, and to showcase successful stories that may benefit others in the field.
Sub-topics
– Machine learning for mixed integer programming
– Machine learning for Makov decision process
– Reinforcement learning
– Energy management
– Autonomous driving
– Manufacturing
– Logistics
– Smart grid
Organizers
– (Samuel) Qing-Shan Jia, Professor, Tsinghua University, China. E-mail: jiaqs@tsinghua.edu.cn
– Bing Yan, Assistant Professor, Rochester Institute of Technology, USA. E-mail: bxyeee@rit.edu
– Bengt Lennartson, Professor, Chalmers University of Technology, Sweden. E-mail: bengt.lennartson@chalmers.se
– Maria Pia Fanti, Professor, Polytechnic University of Bari, Italy. E-mail: mariapia.fanti@poliba.it
Endorsement by the IEEE RAS technical committee on Machine Learning for Automation (https://www.ieee-ras.org/machine-learning-for-automation)
CODE: 276fd
The constant increase of urban mobility over the past decades has improved the overall quality of life around the world. At the same time, when it comes to urban environments, various problems have been arising such as traffic congestion, collisions, and pollution. Consequently, continuous efforts are required to optimize traffic flow, reduce travel time, decrease fuel consumption, and prevent collisions.
A challenge to contribute to traffic flow optimization in critical areas, such as intersections, is being recently given by the advent of Connected, Cooperative and Automated Mobility (CCAM). The capability of Connected, Cooperative and Automated Vehicles (CCAV) to exchange data by a Vehicle-to-Vehicle (V2V) or Vehicle-to- Infrastructure (V2I) makes it possible to implement different classes of optimization tasks and therefore plan routes and schedule crossing times based on priorities, prevent collisions, and ultimately improve traffic streams. The goal is providing benefits to all citizens by implementing a full integration of CCAM services in the transport system by guaranteeing safety (i.e., reducing the number of road accidents caused by human error), reducing transport emissions and congestion by smoothening traffic flow, and avoiding unnecessary trips), ensuring inclusive mobility and good access for all.
The aim of this special session is to propose innovative solutions and tools supporting the technological advancements, facilitating the implementation of innovative CCAM technologies and systems for passengers and goods, develop, implement and demonstrate innovative services for connected and automated vehicles, infrastructures and users. Among the other, Artificial Intelligence, Digital Twin, Simulation and High-Definition Maps, AI approaches (Machine Learning and Deep reinforcement Learning) are the most challenging enablers for CCAM services.
Sub-topics
– Autonomous vehicles
– Electric vehicles
– Traffic Management
– Artificial intelligence (Machine learning and deep reinforcement learning)
– Digital Twin
– Optimization and optimal control
– Logistics
– Simulation
– 5G and 6G network supports
Organizers
– Maria Pia Fanti, Full professor, Polytechnic University of Bari , Italy. E-mail: mariapia.fanti@poliba.it
– Christos G. Cassandras, Distinguished Professor of Engineering, Boston University, USA, E-mail: cgc@bu.edu
– Sebastién Faye, 6G Technology & Innovation Line Manger, Luxembourg Institute of Science, Luxembourg, E-mail:sebastien.faye@list.lu
CODE: eq5e5
Driven by rapid advancements in digital systems, communication technologies, and sensing devices, energy systems, and buildings are evolving into intricate, multi-layered, and distributed structures. Energy systems and buildings are thus becoming prominent multi-agent networked systems integrating heterogeneous entities such as energy sources, energy storage, microgrids, grid networks, and electric vehicles. Within these systems, agents may collaborate to optimize overall performance or compete for shared resources.
The distributed nature of these systems highlights the inadequacy of traditional centralized control and optimization strategies. Limitations, such as vulnerability to failures, costly communications and computations, and a lack of flexibility and scalability, underscore the need for tailored noncentralized algorithms for these systems.
This special session aims to address the challenges and opportunities of noncentralized approaches arising from the characteristic nature of these systems. We invite original articles from scholars and practitioners, presenting theoretical concepts and practical approaches.
Sub-topics
– Advanced noncentralized methods for complex energy systems and buildings.
– New theories and methods for noncentralized control and optimization (pertaining to convergence, optimality, stability, and robustness) with applications to energy systems and buildings.
– Approaches coping with uncertainties arising from renewable energy sources and loads.
– Coordination mechanisms for optimizing energy consumption and resource allocation in energy systems and buildings.
– Human-centric noncentralized control considering user preferences and behaviors.
– Integration of machine learning in noncentralized methods for enhanced adaptability of energy systems and buildings.
– Noncentralized resilience strategies in the face of cyber-physical attacks or failures to energy systems and buildings.
– Real-time communication protocols on noncentralized control and optimization for energy systems and buildings.
Organizers
– Raffaele Carli, Assistant Professor, Politecnico di Bari. E-mail: raffaele.carli@poliba.it
– Paolo Scarabaggio, Assistant Professor, Politecnico di Bari. E-mail: paolo.scarabaggio@poliba.it
– Sergio Grammatico, Associate Professor, Delft University of Technology. E-mail: grammatico@tudelft.nl
– Carlos Ocampo-Martinez, Associate Professor, Universitat Politècnica de Catalunya, E-mail: carlos.ocampo@upc.edu
– Nicanor Quijano Silva, Full Professor, Universidad de los Andes. E-mail: nquijano@uniandes.edu.co
CODE: p3p5j
Semiconductor manufacturing is one of the most complex manufacturing processes. Due to the increased use of new technologies, the global economy is significantly impacted by the semiconductor industry. Competition in the semiconductor sector is fierce. As a result, the semiconductor industry needs to continually reinvent itself and be resourceful at all decision levels. Efficient design, analysis, and operation of semiconductor wafer manufacturing facilities and corresponding supply chains are essential.
Various factors can disrupt certain chains of the production networks. For example, the global supply chain has been disrupted by the Covid-19 pandemic which spreads around the world, resulting, for instance, in chip shortage. All this has an impact on the production (product quality, supply, etc.). Subsequently, effective decisions must be taken to conduct production operations at different levels of the supply chain links while addressing disruptions.
To ensure reliable results and reduced operational costs, highly automated manufacturing systems are used to carry out operations and make millions of decisions per day. This requires planning and scheduling methods in manufacturing execution systems (MES) and in logistics/supply chain management tools to support their automated operation. As new trends such as sustainable production, cloud computing, and Industry 4.0 emerge, they also need to be addressed.
The development and application of planning and scheduling methods for these high-cost systems and supply-chains are critical elements in improving their operations. The purpose of the proposed session is to highlight cutting-edge research on semiconductor manufacturing planning, scheduling, and supplychain management.
Sub-topics
– network planning in semiconductor supply chains
– demand planning for the semiconductor domain
– capacity planning and master planning for semiconductor supply chains
– operational planning approaches in the semiconductor domain
– reference models for semiconductor supply chain planning and control
– (automated) negotiation approaches
– models for the interactions between production and development
– novel scheduling approaches for semiconductor manufacturing
– flexibility and robustness in planning and scheduling
– digital twins for semiconductor manufacturing
– machine learning and other artificial intelligence approaches and tools such as ontologies for planning and scheduling.
Organizers
– Lars Mönch, Prof., University of Hagen, Germany. E-mail: Lars.Moench@fernuni‐hagen.de
– Claude Yugma, Prof., Mines de Saint-Etienne, France. E-mail: Yugma@emse.fr
CODE: x33nr
System of Systems (SoS) is becoming increasingly important in engineering due to its flexibility in different situations while maintaining acceptable performance. This is mainly achievable through distributed control techniques that improve system responsiveness, even in adverse conditions. Distributed control, based on decentralized principles, reduces the risk of failures, enhancing reliability through continuous communication among agents. The proposed special session aims to gather recent findings in the SoS field, with a focus on resilience analysis for new research directions and maintaining high standards in desired behavior despite disturbances, as well as new methods for identifying and recovering from faults.
In addition to the mentioned topics, this special session will also cover the vital areas of traffic systems and biomedical systems in the context of SoS. The exploration of how SoS principles can be applied to improve traffic flow, reduce congestion, and enhance overall transportation efficiency will be undertaken using distributed control techniques. Moreover, the session will investigate how SoS concepts can be employed in biomedical systems, addressing challenges such as real-time monitoring, adaptive control, and fault tolerance in healthcare setups. By including these key domains, the special session aims to encourage discussions and advancements that broaden the application of SoS principles across diverse and intricate systems.
Sub-topics
– Distributed control approaches
– Autonomous system control
– Emergent behaviour in system of systems
– Control techniques in sustainable systems
– Vehicle platooning
– Automotive
– Smart manufacturing systems
– System of Systems Engineering
Organizers
– Alessandro Bozzi, Research fellow, University of Genoa. E-mail: alessandro.bozzi@edu.unige.it
– Simone Graffione, Research fellow, University of Genoa. E-mail: simone.graffione@edu.unige.it
– Elinda Kajo Mece, Full Professor, Polytechnic University of Tirana, E-mail: ekajo@fti.edu.al
– Roberto Sacile, Full Professor, University of Genoa. E-mail: roberto.sacile@unige.it
– Ilir Shinko, Assistant Professor, Polytechnic University of Tirana, E-mail: shinko@fti.edu.al
– Enrico Zero, Assistant Professor, University of Genoa. E-mail: enrico.zero@dibri.unige.it
CODE: d28pn
Many systems, such as automated control systems, smart grids, biology, economics, finance, and autonomous systems, generate time series data that have a high number of variables but a low dimension of underlying dynamics. This makes the data analysis difficult and challenging, as finding the latent dynamic variables and structures that govern the observed dynamics in the data is essential for systems modeling, process understanding and data visualization. Reduced-dimensional dynamic data analytics aims to address this challenge to extract the essential dynamics information from data. This problem involves understanding the complex interactions between high dimensionality, reduced-dimensional dynamics, and domain-specific features that are useful for prediction, interpretation, diagnosis, and decision-making.
Reduced-dimensional dynamic data analytics is a fast-growing area to analyze high-dimensional data with low-dimensional dynamics. For data with low-dimensional dynamics, some linear combinations of the variables are white noise even though each observed variable is serially correlated. Box and Tiao (1977) first introduce canonical analysis of the vector autoregressive (VAR) residuals to find combinations that lead to predictable latent variables. Engle and Watson (1981) interpret the extracted dynamic latent variables as market co-moving factors. Dynamic factor models (DFM) have been extensively studied in econometrics to extract dynamic factors in a reduced dimensional subspace (Pan and Yao, 2008; Lam and Yao, 2012; Pena et al., 2019; and Gao and Tsay, 2021), while dynamic latent variables are recently developed to extract explicit and compact latent dynamic models such as latent VAR models (Dong and Qin, 2018; Qin et al., 2020; Qin, 2022).
This special session aims to present the latest advances and applications of reduced-dimensional dynamic data analytics, covering general latent dynamic methods, as well as domain-specific approaches that address challenging problems in various areas. The session welcomes contributions that highlight the unique characteristics and analytical needs of different types of high-dimensional dynamic data, such as spatio-temporal, multi-modal, real-time, and distributed network data. The session also encourages contributions on relevant developments in machine learning such as slow factor analysis (SFA). The session invites submissions that explore this exciting field and its applications to cope with the increasing dimensions of dynamic data with low-dimensional dynamics in the real world. An expected outcome of the session is to share new methodology developments and demonstrate the practical use of latent variable analytics in specific domains to provide valuable insights into the benefits and opportunities.
Sub-topics
– General models and methods for reduced-dimensional dynamic data analytics
– Efficient algorithms for reduced-dimensional dynamic data analytics
– Dynamic factor modeling
– Data-driven latent dynamic modelling, prediction, and fault diagnostics
– Spatio-temporal analysis of latent dynamic data
– Multi-modal latent dynamic data analytics
– Distributed latent dynamic data analytics
– Machine learning for latent dynamic data analytics
– Decision-making with latent dynamic models
– Applications in the process automation, intelligent transportation, smart grids, social networks, economics, finance, ecology, biology, etc.
Organizers
– S. Joe Qin [Co-Lead Organizer], Wai Kee Kau Chair Professor of Data Science, Institute of Data Science, Lingnan University, Hong Kong. Email: joeqin@ln.edu.hk
– Yining Dong [Co-Lead Organizer], Assistant Professor, School of Data Science, City University of Hong Kong. Email: yining.dong@cityu.edu.hk
– Lishuai Li, Associate Professor, School of Data Science, City University of Hong Kong. Email: lishuai.li@cityu.edu.hk
– Yanfang Mo, Postdoc, Hong Kong Institute for Data Science, City University of Hong Kong. Email: yanfang.mo@cityu.edu.hk
CODE: r41wu
Systems Man and Cybernetics and Robotics and Automation Societies both encourage interdisciplinary
approaches in engineering that spread among various fields, such as computer science, control systems, electrical engineering, mathematics, mechanical engineering, system engineering, human systems, human organizational interactions, cybernetics, communication and control across humans and machines. This Special Session particularly focuses on human-machine interaction, for which is it is of paramount importance to ensure safety of human beings and security of machines. In fact, improper control of machines might cause accidental harms to operators; and also safety breach can lead the machine to unsafe states and cause damage to the surrounding environment, including operators. The main aim of this session is to collect contributions on the most recent cross-disciplinary techniques to develop Safe and Secure Human-Machine Interaction. Thus, enabling the advancement of modelling, control, and information processing in various related application fields, such as manufacturing, logistics, smart mobility, aerospace, smart cities, networks, etc. In addition, this session is devoted also to the celebration of the Italian SMC Day 2024 by collecting some of the most significant and advanced research and contributions of SMCS and/or RAS Members on the topic. Original articles are welcome, reporting on theories and methods for Safe and Secure Human-Machine Interaction and addressing system and control engineering topics.
Sub-topics
– Human-centered automation
– Automation in manufacturing
– Networks of sensors and actuators
– Supervisory control
– Cyber-physical systems
– Cyber-physical human systems
– Mobile robotics
– CyberaUacks and Cybersecurity
– Intrusion detection systems
– Fault diagnosis and prognosis
– Human in the Loop
– Internet of Things
– Social networking
– Activity recognition
– Wearable sensors
– Machine Learning
– Situation Awareness
– Brain-Computer Interface
Organizers
– Laura Giarrè, Full Professor, Università di Modena e Reggio Emilia – Italy. E-mail: laura.giarre@unimore.it
– Giancarlo Fortino, Full Professor, Università della Calabria – Italy. E-mail: giancarlo.fortino@unical.it
– Graziana Cavone, Assistant Professor, Università degli Studi Roma Tre – Italy. E-mail: graziana.cavone@uniroma3.it
– Giuseppe D’Aniello, Assistant Professor, Università degli Studi di Salerno – Italy. E-mail: gidaniello@unisa.it
CODE: 9tt8e
Autonomous systems represent a key enabling technology for the digital transition. Autonomy can be achieved by properly collecting and processing a large quantity of data, and use them in combination with models to drive actuation and achieve proper functioning. It is thus of critical importance that unexpected deviations from nominal behaviours, due for instance to faults, can be detected and quickly addressed before degenerating into a total system failure, which may cause both economical and human losses. For instance, an unmanned aerial vehicle experiencing a fault should be able to return home without crashing to the ground, or a faulty industrial robot should be able to slow down before causing harm to a human operator.
The challenge of increasing the safety of autonomous systems can be addressed in different ways, and this special session is focused on the operational phase, where the occurrence of a fault is addressed by means of Fault Detection and Isolation (FDI), Fault Diagnosis (FD), Fault-Tolerant Control (FTC) and Fault Prognosis (FP). The special issue is open to both model-based and data-driven approaches, as well as their combination to cope with robustness problems which typically arise in the application domains. Moreover, contributions which use diagnostic and prognostic information to trigger maintenance when actually needed, in the so called Condition Based Maintenance (CBM) and Predictive Based Maintenance (PBM) paradigms, are welcome.
The projected outcome of the special session is to collect relevant contributions in the field of safety in autonomous systems, to provide an understanding of the open problems as well as future directions on how to bridge the gap between theory and practice for increasing autonomy and safety of systems of the future.
Sub-topics
– Fault Detection and Isolation (FDI)
– Fault Diagnosis (FD)
– Fault-tolerant Control (FTC)
– Fault-prognosis (FP)
– Robustness issues in Fault Detection and Diagnosis
– Robustness issues in Fault-tolerant Control
– Integration of model-based and data-driven methods in Fault Diagnosis
– Integration of model-based anda data-driven methods in Fault Tolerant Control
– Applications of fault diagnosis to autonomous systems
– Application of fault tolerant control to autonomous systems
– Condition Based Maintenance (CBM)
– Predictive Based Maintenance (PBM)
Organizers
– Alessandro Freddi, Associate Professor, Università Politecnica delle Marche (Ancona, Italy). E-mail: a.freddi@univpm.it
– Alessandro Baldini, PostDoc Researcher, Università Politecnica delle Marche (Ancona, Italy) . E-mail: baldini@univpm.it
– Riccardo Felicetti, PostDoc Researcher, Università Politecnica delle Marche (Ancona, Italy) . E-mail: r.felicetti@univpm.it
– Silvio Simani, Associate Professor, Università degli Studi di Ferrara (Ferrara, Italy). E-mail: silvio.simani@unife.it
– Mirko Mazzoleni, Assistant Professor, Università degli Studi di Bergamo (Bergamo, Italy). E-mail: mirko.mazzoleni@unibg.it
CODE: yp9d7
Smart manufacturing, in the era of Industry 4.0, leverages advanced technologies such as the Internet of Things (IoT), artificial intelligence (AI), data analytics, robotics, and cyber-physical systems. The objective is to create intelligent, interconnected, and automated manufacturing systems, thus leading to enhanced productivity, improved quality control, reduced downtime, and increased flexibility. Simultaneously, sustainable manufacturing has gained considerable attention as a means to address the environmental, social, and economic challenges associated with conventional manufacturing. Sustainable manufacturing focuses on minimizing resource consumption, reducing waste generation, optimizing energy usage, and adopting eco-friendly production processes. There is a growing inclination among customers and markets towards digital, customized, and flexible solutions with a reduced environmental impact. This inclination aligns with the central concept of Industry 5.0, which complements the Industry 4.0 approach by placing research at the forefront of the transition to a sustainable, human-centric, and resilient industry. The integration of smart and sustainable manufacturing is pivotal, holding the potential to revolutionize the environmental and economic impact of the manufacturing industry.
This session will be an excellent opportunity for networking, collaboration, and knowledge exchange among industry experts, academics, and professionals who are passionate about advancing the field of manufacturing towards a more intelligent and sustainable future.
Sub-topics
– Sustainable and Resilient Manufacturing Systems: Strategies and Technologies
– Energy-efficient and Eco-Friendly Production Processes
– Digital Twin Technologies for Smart and Sustainable Production
– Innovations in Human-Robot Collaboration
– Circular Economy Practices in Manufacturing
– Life Cycle assessment in Smart and Sustainable Manufacturing
– Advancements in Flexible Manufacturing System Modeling and Control
– Intelligent Decision-Making for Sustainable Production Systems
– Human-Machine Interfaces and Ergonomics in Sustainable Manufacturing
– Case Studies on Successful Implementation of Sustainable Manufacturing Practices
– Innovative Materials for Eco-Friendly Manufacturing Processes
– Industry 5.0: Bridging the Gap between Technology and Sustainability
Organizers
– Qing Chang, Professor, School of Mechanical and Aerospace Engineering, University of Virginia. Email: qc9nq@virginia.edu
– Congbo Li, Professor, College of Mechanical and Vehicle Engineering, Chongqing University. E-mail: congboli@cqu.edu.cn
– Wei Wu, Assistant Professor, College of Mechanical and Vehicle Engineering, Chongqing University. E-mail: bravew@cqu.edu.cn
– Feng Ju, Associate Professor, School of Computing and Augmented Intelligence, Arizona State University. Email: fengju@asu.edu
– Nicla Frigerio, Assistant Professor, Department of Mechanical Engineering, Politecnico di Milano. E-mail: nicla.frigerio@polimi.it
CODE: 2845n
Soft robotics aims at solving a wide range of automation challenges using a soft, sensorised, active and programmable materials. The goal of this special session is to connect researchers working on cutting-edge soft robotics technologies and the scientific community developing automation 5.0.
Soft robotics technologies excel in tasks that require contact with the human body or with delicate objects, thanks to the use of compliant components. Textile-based exoskeletons for haptics, rehabilitation and muscle support are getting lightweight, comfortable to wear and minimally invasive. Robotic companions for human assistance made partially with soft materials can have safe, smooth interactions with humans, and a reassuring appearance reminding Big Hero’s Baymax. Soft grippers can pick up raspberries as delicately as human workers.
Furthermore, the compliance of soft materials makes these robots versatile. Soft grippers can typically grasp a wide variety of objects of variable and complex shapes thanks to soft fingers that conform to the shape of the object. Soft manipulators can bend virtually at any point, shrink, elongate, and even change their stiffness based on the task requirements. Crawlers, bionic insects and vine robots can squeeze through tight openings, inspecting even the most remote and dangerous locations.
The projected outcome of this special session is to bridge soft robotics technologies with the needs of tomorrow’s automation tasks. We expect to kickstart communication and collaborations between groups working in soft robotics and groups leading automation research.
Automation 5.0 will require direct interactions with humans, a high degree of flexibility and the ability to operate in unstructured environments. Thanks to a unique combination of versatility, safe contacts with humans, wearability, and toughness, soft robotic technologies will contribute to the wide spreading of ubiquitous automation.
Sub-topics
– Soft grippers
– Soft robots for exploration, inspection, and maintenance
– Wearable soft robotics
– Soft robotics for haptics and HCI
– Soft sensors and soft actuators
– Soft robotics for agriculture
– Soft manipulators
– Modeling and control of soft robots
– Soft-matter fluidics
Organizers
– Vito Cacucciolo, Associate Professor, Politecnico di Bari (Italy). E-mail: vito.cacucciolo@poliba.it
– Francesco Giorgio-Serchi, Lecturer and Chancellor’s Fellow, The University of Edinburgh (UK). E-mail: F.Giorgio-Serchi@ed.ac.uk
– Federico Renda, Associate Professor, Khalifa University (UAE). E-mail: federico.renda@ku.ac.ae
CODE: 5rf2s
In recent years, there has been a transformation in Space activities. While in the past, only a few companies contributed to the different Space agencies activities, now hundreds of companies are developing their business based on activities in Space going by the hands of Space Agencies or on their own. This is based on the broad number of potential operations that could be performed thanks to the latest advancements in the autonomy of robotics systems in Space. Operations such as in-orbit refuelling, repairing/maintenance of other satellites, assembly of large structures, or even manufacturing in orbit are opening a lot of possibilities for companies to develop new businesses and to academia to focus on new challenges. In the case of planetary activities, something similar is happening. The recent discoveries of water-ice in the polar regions of the Moon and the development of different processes of the Moon-dust (regolith) to obtain Oxygen, and metal-oxides or use it as a construction material are calling to develop further industry and academia technologies and the fundamental and applied research.
In this sense, autonomy will play a crucial role in both space exploration and exploitation. In exploration missions, autonomy is required to increase scientific return, avoiding basic teleoperation from Earth, which is impossible on remote planets due to communication delays and limited timeslots. In the case of orbital robotics, even if the operations are carried out on orbit of Earth, autonomy will be required to perform tasks that require high precision, e.g. docking and manipulation.
Therefore, this special session pursues to place value on the research activities that are carried out by different research institutions and companies on the field of space autonomy, in both orbital and planetary scenarios, making emphasis on how this autonomy can be applied to solve particular problems that are found in space.
Sub-topics
– Planetary exploration
– Multi-robot Collaboration in Space
– Robotic Manipulation in Space
– Orbital servicing
– Rendezvous and docking of satellites
– In-space assembly and/or manufacturing
– In-situ resource processing and utilization
– Ground facilities for Space high-fidelity representation
– Sensing and perception in Space
Organizers
– Miguel Olivares-Mendez, Professor and head of the Space Robotics (SpaceR) Research Group. University of Luxembourg, SpaceR research Group. Email: miguel.olivaresmendez@uni.lu
– David Rodríguez Maranez, Research Scientist, EPFL Advanced Quantum Architecture (AQUA) Lab. Email: david.rodriguez@epfl.ch
– Carlos Pérez del Pulgar, Assoc. Prof., University of Malaga, Space Robotics Lab. Email: carlosperez@uma.es
– Dimos V. Dimarogonas, Professor, Head of the Division of Decision and Control Systems, KTH Royal Institute of Technology. Email: dimos@kth.se
– Pedro Roque, PhD Student, Division of Decision and Control Systems, KTH Royal Institute of Technology. Email: padr@kth.se
– Kentaro Uno, Assistant Professor, Tohoku University, Japan.
CODE: 8qe34
In the last decade, the smart cities and industry 4.0 paradigms have experienced remarkable growth and several research programs have been carried out to show the potential and the positive impact provided by smart technologies aimed at increasing the efficiency and the sustainability of the urban and industry environments.
The integration of Smart Cities and Industry 4.0 (even the new Industry 5.0 paradigm) represents a transformative synergy, converging urban development with advanced industrial processes.
Moving from these premises, this special session aims at exploring the multifaceted connection between these two paradigms, emphasizing the mutual benefits derived from their combination involving the seamless incorporation of technologies such as the Internet of Things (IoT), artificial intelligence (AI), and data analytics into both urban and industrial landscapes.
Realistic research initiatives in this area requires a multidisciplinary framework involving experts in network systems analysis, design and control, fault tolerance and diagnosis, data analysis and communications.
Sub-topics
– Integration of Industry 4.0 Technologies in Smart City Infrastructure
– Automation and Control Solutions for Sustainable Urban Development
– Cyber‐Physical Systems in Smart Cities
– Data Analytics for Smart City Decision‐Making in Industry 5.0 Era
– Security and Privacy Concerns in the Convergence of Smart Cities and Industry 4.0
– Human‐Centric Design in Industrial Automation for Urban Living
– Interoperability of IoT Devices and Sensors in Smart Cities
– Role of Artificial Intelligence in Enhancing Urban Control Systems
– Resilient Infrastructure: Control Strategies for Smart Cities
– Digital Twin Applications for Urban Planning and Industry 4.0
– Sustainable Transportation Systems with Automation and Control
Organizers
– Gianfranco Gagliardi, Researcher, Università della Calabria. E‐mail: g.gagliardi@dimes.unical.it
– Francesco Tedesco, Associate Professor, Università della Calabria. E‐mail: ftedesco@dimes.unical.it
CODE: yy574
In intelligent systems, big data analytics (BDA) provide meaningful insights for production decision making by processing and analysing massive amounts of industrial data, which reduces costs and improves operational efficiency. With the development of data science and artificial intelligence, BDA have been greatly improved to effectively mine both structured and unstructured industrial data that hides knowledge and insights to enable intelligent systems.
Nevertheless, there still exists a significant gap in achieving trustworthy intelligent systems due to the susceptible and inexplicable algorithms and the imbalance industrial data. It is difficult for staff to intuitively understand the reasons behind decisions made by inexplicable algorithms. Moreover, data-driven algorithms trained by limited and imbalance industrial data cannot automatically adapt with changes in dynamic engineering scenarios and lead to decision-making bias. This calls for trustworthy BDA technologies to improve the reliability, stability, and interpretability of intelligent systems.
To this end, as an emerging and promising research topic, this special session is dedicated to present the state-of-the-art and methodologies, tools, systems, and practical applications to enable the readiness and realization of trustworthy BDA.
Sub-topics
– Trustworthy BDA theories for intelligent systems
– Trustworthy BDA for automated modeling, simulation, and control
– Trustworthy BDA-enabled adaptive automation systems
– Trustworthy BDA-based engineering applications
– Trustworthy BDA-enabled automated production quality control
– Trustworthy BDA-aided automated fault diagnostics and prognostics
– Trustworthy BDA-enabled automated planning and scheduling
– Trustworthy BDA-enabled automated decision making and process optimization
– Trustworthy BDA-enabled sustainability and green automation
Organizers
– Junliang Wang, Associate professor, Donghua University. E-mail: junliangwang@dhu.edu.cn
– Xinyu Li, Professor, Huazhong University of Science & Technology. E-mail: lixinyu@mail.hust.edu.cn
– Wei Qin, Associate professor, Shanghai Jiao Tong University. E-mail: wqin@sjtu.edu.cn
– Jie Zhang, Professor, Donghua University. E-mail: mezhangjie@dhu.edu.cn
– Xiaoou Li, Professor, CINVESTAV-IPN. E-mail: lixo@cs.cinvestav.mx
Contact
For any inquiries please contact the Special Session Chairs.
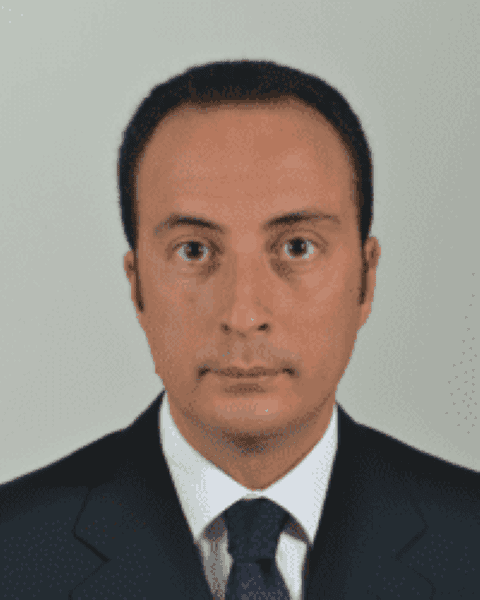
Raffaele CARLI
Polytechnic of Bari
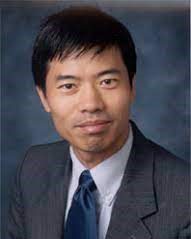
Mengchu ZHOU
New Jersey Institute of Technology
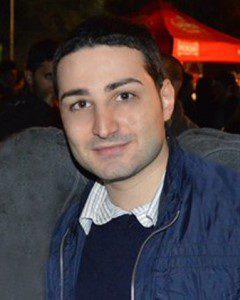
Claudio SAVAGLIO
University of Calabria
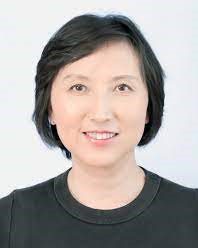
Ying (Gina) TANG
Rowan University
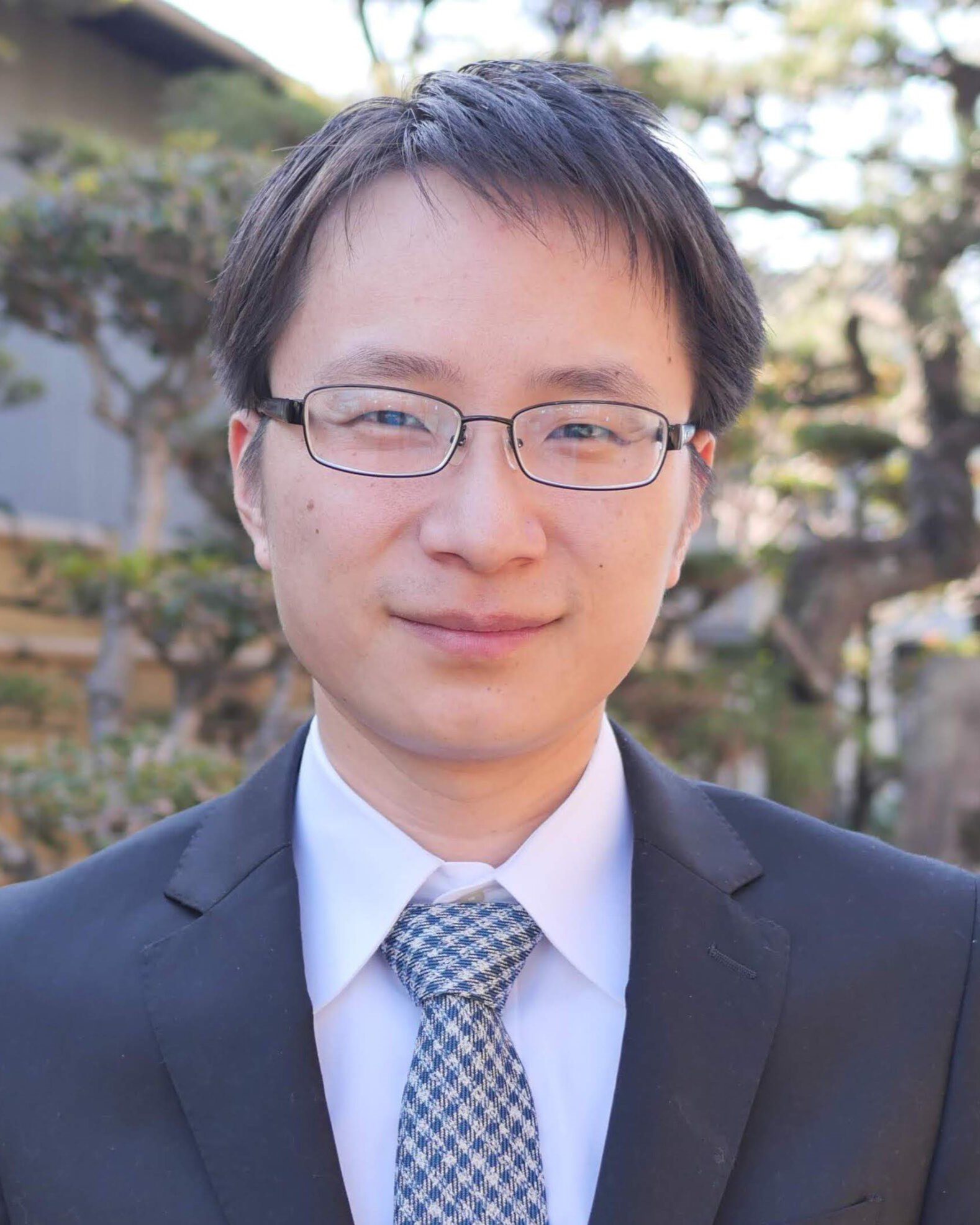
Kai CAI
Osaka Metropolitan University